Medical professionals seek better ways to streamline healthcare deliveries and improve patient outcomes. In recent years, there have been growing opportunities for AI to play more prominent roles in supporting their causes. At a glance, AI is poised to bring transformational changes to the healthcare industry. By 2030, the global market for healthcare AI is predicted to hit $187.95 billion, marking a 37% CAGR from 2022.
However, ethical and privacy concerns require stricter and more transparent AI governance in healthcare applications. To encourage AI usage, stakeholders need to follow a robust ethics of AI in healthcare framework that balances AI's benefits and mitigates ethical challenges. Yet, known AI limitations and risks shouldn’t obscure its potential to improve healthcare deliveries.
As Uptech’s co-founder and tech lead, I’ll draw from our experience building several generative AI apps and expand on their implications when applied to healthcare solutions. More importantly, I’ll guide you through establishing an AI governance framework to support your healthcare product.
If you’re keen on leveraging the opportunities that AI brings, read on and learn how to implement safer, reliable, and trustworthy AI solutions.

Ethical Concerns and Governance in Healthcare AI
Medical providers face ethical issues with artificial intelligence in healthcare – even before AI was widely implemented. However, recent advancements in generative AI exacerbated such concerns, requiring key stakeholders to revisit efforts to safeguard patient’s interests better. Advanced AI healthcare technologies, particularly those fuelled by deep learning algorithms, lack transparency and observability. For example, doctors face difficulties explaining how AI reaches a conclusion when analyzing MIR scans.
Patients rightfully expect fair and equal treatment throughout their healthcare experience. Such expectations might be compromised when AI demonstrates societal bias. Recently, a study revealed that millions of patients are affected by algorithmic bias that favors specific demographics based on their earnings. Generative AI, which trains on massive datasets, can inherit bias influencing their inference in medical use cases.
Besides ensuring fair treatment, healthcare providers grapple with data challenges when training, deploying, and scaling medical AI solutions. Unlike non-critical AI products, medical AI relies on medical datasets for domain-specific tasks. Training and operating the AI models involve moving massive volumes of sensitive data across storage. Securing these data is essential to sustain patient trust and comply with healthcare regulations.
Therefore, healthcare providers seek a framework to regulate ethical issues with AI in healthcare. They need to formulate policies, guidelines, and standard operating procedures to apply AI more confidently and responsibly. More importantly, AI governance imposes responsibilities to all parties along the patient care workflow.
Why healthcare provider CIOs need to establish AI governance

Generative AI is continuously evolving, uncovering new opportunities and unknown challenges that bring healthcare institutions into uncharted territories. This underscores the importance of the ethics of AI in healthcare before introducing new AI technologies. And the responsibility falls on CIOs in healthcare institutions.
I share several reasons why such efforts are necessary below.
Mitigating data risks
As mentioned, healthcare institutions face problems with AI in healthcare, such as adversarial cyber threats that might compromise their ability to provide optimal treatment. CIOs need to devise ways, such as encryption and authentication, to secure the large volumes of data that AI uses. Such efforts also help hospitals and clinical facilities to comply with industry regulations, such as HIPAA and GPDR.
Improving AI predictions
Establishing machine model training guidelines as part of the governance framework helps improve AI performance. While AI models offer powerful predictive capabilities to aid medical decisions, they are only as accurate as the datasets they train. CIOs must ensure that AI products applied in medical facilities are trained with quality datasets that fairly represent the patient demographics.
Ensuring accountability
Deep learning AI models lack observability because of their multiple layers of hidden neural networks. In healthcare, CIOs should proactively distribute responsibility and promote accountability among medical workers and AI experts. They ensure each party understands their role in securing and administering AI-generated data to augment medical-related decisions.
Accelerating positive business outcomes
Private healthcare facilities strive to balance revenue growth, profitability, and quality patient care. With a governance framework, CIOs can support healthcare institutions’ pursuit of modern AI technologies while staying aligned with organizational goals. Moreover, the framework minimizes risks as healthcare providers take pioneering steps in AI. For example, CIOs can prioritize budgets and resources to support AI initiatives with clear and quantifiable policies.
7 Steps to Establish AI Governance in Healthcare
AI will inevitably integrate itself into clinical workflows. The question is when and whether healthcare providers can devise a sustainable framework to enforce governance. These steps provide a solid foundation to implement a fair, autonomous, and inclusive AI platform in the healthcare industry.
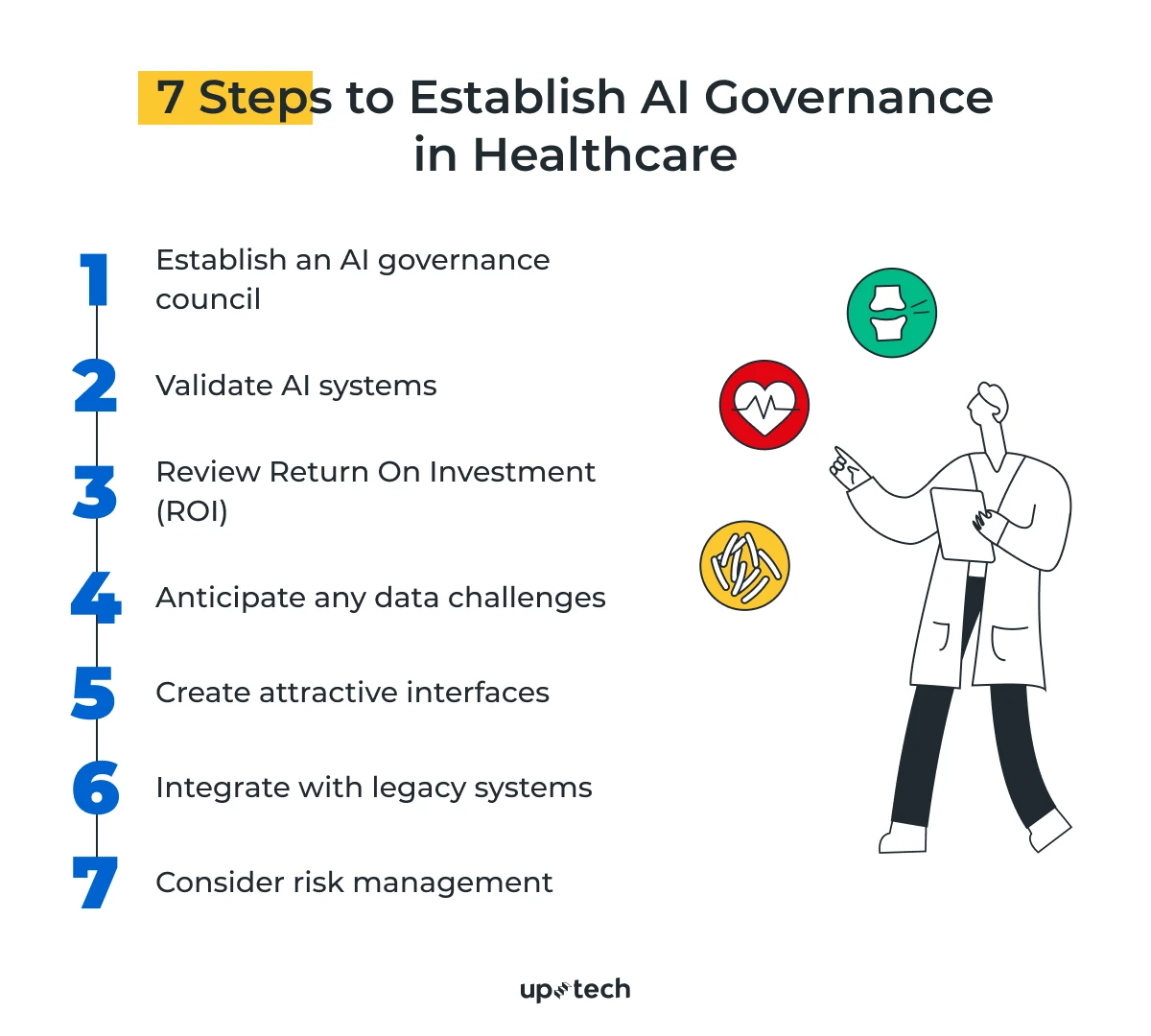
1. Establish an AI governance council
The AI governance council consists of authoritative parties with a vested interest in the risks and rewards that AI brings. They include medical experts, IT teams, security officers, and AI specialists responsible for advising and navigating the institution through AI’s complexities. For example, the council provides recommendations for:
- Mitigating data risks.
- Achieving compliance.
- Drafting AI usage policies for clinicians and patients.
Pro-tip - It’s essential that the entire organization share a common understanding of the risks, challenges, and benefits of medical AI technologies.
2. Validate AI systems
Do your due diligence before procuring AI solutions for healthcare applications. Each AI model has varying performance, with some more suited for medical use cases than others. When choosing an AI platform, ask probing questions, such as:
- How will the AI system automate or streamline existing clinical processes?
- Does the AI system exhibit significant bias or inaccuracies? If so, what are the mitigative measures?
- Is the AI product compliant with healthcare regulations?
Pro tip: Preferably, deploy AI systems designed for a specific clinical task, such as medical record summarization. Then, scale or deploy additional AI tools across other use cases when the initial AI system proves stable.
3. Review Return On Investment (ROI)
Procuring medical AI solutions is a financial investment that key stakeholders hope will bear positive returns. While the direct impact on revenue growth provides confidence in supporting the decision, you should also consider other qualitative influences.
For example,
- Will introducing medical AI lead to job loss among medical workers?
- Can the AI product automate medical research or advance existing treatment techniques?
- Does using AI reduce wastage and contribute to environmentally sustainable goals?
Pro-tip: Don’t neglect AI’s impact on patient trust and your institution's reputation.
4. Anticipate any data challenges
Deep learning AI systems strictly adhere to the adage ‘garbage in, garbage out’. Inconsistencies and anomalies in its training dataset will impact real-life system performance. For example, if trained with questionable datasets, the AI system might falsely categorize pathological symptoms. So, be mindful of these concerns when applying AI in healthcare environments.
- Data quality. Curate data from diverse but relevant sources. Engage medical experts to collaborate with machine learning teams to clean, label, and prepare the training datasets.
- Data scarcity. Take measures to augment the training dataset if the development team can’t secure enough data to train the model.
- Data security. Apply security best practices to improve the data pipeline’s resilience to external threats.
Pro-tip: Determine the data types you need to develop and operate the AI system. Then, use appropriate tools to collect, store, and load the data to feed the AI system.
.webp)
5. Create attractive interfaces
Remember that doctors, medical staff, and patients are the eventual users of the deployed AI system. Therefore, the governance guideline shall include specifications and requirements for the system’s user interface. From onboarding to routine usage, all users must feel at ease with the controls, descriptions, navigation, and visuals the AI app provides.
For example, the AI platform should:
- Abstract the complexities of the underlying AI technologies.
- Provide users with an intuitive interface to access advanced features.
- Display on-screen guidance when necessary to ease the learning curve.
Pro-tip: Collect feedback from medical users and patients to ensure you enlist the proper UI requirements in the governance framework.
6. Integrate with legacy systems
Healthcare AI platforms must ingest data from existing systems to predict or generate data-driven recommendations. The challenge lies in integrating with systems that may need to be compatible or upgradable to meet AI data requirements. Moreover, some healthcare technicians, radiologists, and clinicians are accustomed to legacy systems, making switching to more advanced AI platforms more challenging.
So, consider these integration challenges and find ways to bridge the technological gaps.
- Can the AI system fit seamlessly into existing medical workflows? Is additional process modification needed?
- Does the AI platform support clinical or networking protocols that other equipment uses?
- Is the healthcare facility’s infrastructure capable of supporting AI systems and intense data processing?
Pro-tip: There is no one-size-fits-all AI solution. Expect a degree of customization to fit the AI platform with legacy systems.
7. Consider risk management
While AI experts continuously release better deep learning AI models, healthcare providers must manage the risks that come with it. Taking a pragmatic approach when implementing AI allows you to improve healthcare quality while safeguarding patient’s interests. Specifically, pay attention to these risks that could negate the benefits of AI if not appropriately handled.
- Data privacy and compliance challenges that might lead to breaches and hefty penalties.
- Reputational risks could undo the hard work that healthcare providers took to turn them into trustworthy establishments.
- Infrastructural risks could compromise existing medical systems if AI is not correctly integrated or deployed.
Pro-tip: Establish contingency measures in the governance framework for every possible scenario to minimize undesirable impacts.
Summary
AI, particularly deep learning models, holds tremendous potential to transform patient care experiences. Yet, healthcare providers need a robust governance framework to ensure a responsible, transparent, and fair AI experience for patients and medical professionals. CIOs, vital in enabling technological changes, are pivotal in devising appropriate guidelines and procedures for healthcare AI development, deployment, and improvements.
I’ve shared practical steps to create your own AI governance framework for medical institutions. That said, some founders and product managers prefer collaborating with AI development teams with field-proven experience. Doing so allows them to focus on delivering key benefits that impact the clinical process while relying on an experienced partner to mitigate governance risks and ensure ethics of AI in healthcare.
Uptech has built and integrated several generative AI applications in recent months. For example, we built Dyvo, an app that turns selfies into realistic AI-generated avatars. We’ve also developed a mental health app for the US market, which applies stringent data security practices.
Talk to us about supporting healthcare AI systems with a robust governance framework.