AI technologies, especially those with generative capabilities, are influencing all industries. Healthcare is no exception. AI medical image analysis systems are becoming an integral part of disease detection, diagnosis, and treatment.
But AI's impact goes beyond these areas. For instance, generative AI models are widely used to colorize medical images. They add helpful color details that provide additional information for diagnosis, treatment planning, and educational purposes. Paired with 3D modeling, AI is also used in surgical planning and intervention.
At the same time, AI technology is continuously evolving, which complicates efforts to bring such products to the market. Moreover, healthcare is a tightly regulated industry that requires specific knowledge to ensure a compliant product. This begs the question — how to build AI medical imaging analysis software while navigating multiple hurdles along the way?
As the co-founder and AI expert at Uptech, I’ve helped numerous companies with their AI development efforts. We’ve integrated a generative AI virtual assistant to Plai, built an AI summarizer, Hamlet, and released our AI-based social avatar generator, Dyvo AI. With this article, I want to help you unleash your medical AI solutions.
So, read on to learn more about AI in medical imaging and diagnostics and how such diagnostics tools are built.
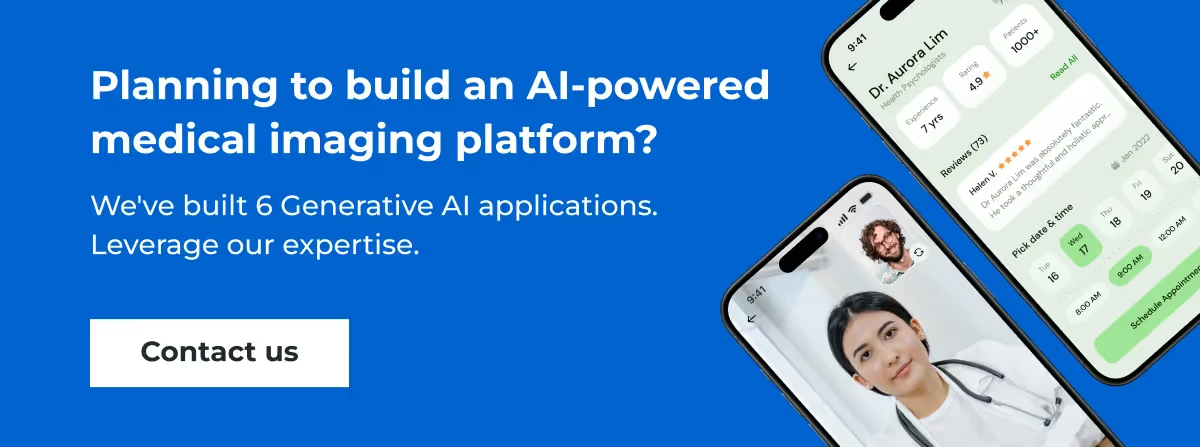
How AI-Based Clinical Image Analysis is Transforming Healthcare
There is no better time for entrepreneurs to invest, innovate, and release their own AI-powered medical imaging tools.
Studies show that AI can reduce diagnostic errors by up to 20%, which provides great potential for patient care improvement. Also, according to Statista, AI has been proven to save physicians 17% of their time in administrative tasks.
In addition to administrative tasks, AI can significantly reduce the time required to diagnose diseases. For instance, AI-driven image analysis tools can process and interpret scans much faster than traditional methods, leading to quicker diagnosis and timely treatment initiation.
So, let’s examine closely how AI is shaping the healthcare domain and what impact it has on medical imaging analysis.
Current challenges faced by the healthcare industry
There would be the need for AI in medical imaging and diagnostics, if the domain had no problems. Chances are you know this, but let me remind you of the key problems that the healthcare sector is facing now.
Misdiagnosis risk. Diagnostic mistakes are some of the most common and harmful medical errors, with between 12 to 18 million Americans experiencing some form of misdiagnosis each year. Some of such mistakes result in deaths and disabilities.
Inefficient workflows. In healthcare, medical imaging workflows often suffer from manual data entry, long processing times, and communication gaps between departments. These issues can cause delays in diagnosis, increased workloads for radiologists, and longer waiting times for patients.
Typically, it takes about 4 to 5 hours to prepare an MRI report. After the MRI scan is completed, the images need to be reviewed by a radiologist, who then prepares a detailed report.
Aging population. Healthcare providers are also struggling with the growing number of aging patients. According to the World Health Organization, in 2019, there were 1 billion people aged 60 years and older. This number is expected to rise to 1.4 billion by 2030 and 2.1 billion by 2050.
Radiologist shortage. Speaking of radiologists, there is a global shortage of these doctors. According to recent data from Statista, there are about 50,000 practicing radiologists in the United States, which has a population of 341,918,869 as of 2024. This means each radiologist is responsible for approximately 7,000 patients.
Data privacy and security issues. There are also significant challenges in healthcare medical imaging related to data privacy. Patient data, including medical images, contains sensitive information that must be protected to comply with regulations like HIPAA and GDPR. Mishandling or unauthorized access to this data can lead to privacy breaches and legal consequences.
And this list of challenges is just the tip of the iceberg. But fret not. AI comes in to make that iceberg disappear or at least smaller.
What is AI medical imaging, and how can it help?
Medical imaging, in general meaning, refers to a variety of approaches and technologies used to diagnose, monitor, or treat medical conditions of the human body.
Medical imaging includes but is not limited to the following modalities:
- Conventional X-rays
- Computed Tomography (CT) scans
- MRI scans
- Sonography, etc.
There is also an international standard for transmitting, storing, retrieving, printing, processing, and displaying medical imaging information called DICOM (Digital Imaging and Communications in Medicine).
Any software that can "analyze" data from medical images is called medical image analysis software. And it must be developed with this standard in mind.
Recently, there has been a wide use of artificial intelligence for medical image analysis purposes.
AI-powered medical imaging platforms use AI techniques like computer vision and deep learning algorithms to analyze data from medical images on a more nuanced level.
Unlike traditional software, which mainly rely on predefined rules and algorithms, AI-based software can:
- learn from large datasets,
- adapt to new information, and
- identify patterns that might not be easily seen or evident through standard analysis.
That said, how does AI solve the issues we discussed earlier?
AI-based clinical image analysis opens the door to more accurate, faster, and better insights into medical imaging data. Physicians use AI-powered medical imaging platforms to detect various medical symptoms, including cardiovascular diseases, cancer, dermatitis, and fractures.
AI also optimizes image acquisition, storage, and retrieval, making workflows more efficient. It can also use predictive analytics to forecast patient volume and resource needs, enabling better operational planning.
For data security, AI offers advanced encryption and anomaly detection to protect patient information. Additionally, AI can anonymize medical images, ensuring privacy while keeping data useful for research.
I suggest that we look into the benefits more precisely.
How does AI medical imaging analysis benefit your business?
Integrating AI capabilities with medical imaging offers patients and healthcare providers wide-ranging benefits. I have rounded up the key ones in this section.

Early disease recognition
Some pathological symptoms might escape manual human inspections because they are subtle and hardly discernable from the imaging data. Deep learning models like convolutional neural networks (CNN) can extract in-depth spatial features from the imaging results and reveal the disease characteristics that are not visible to the human eye. This allows both doctors and patients to be aware of possible symptoms and seek further consultations.
Diagnosis time reduction
Applying AI in medical imaging processing streamlines operational setups and analysis. Instead of manually analyzing radiology scans, doctors can speed up medical diagnoses with the AI scanner's summaries. As such, healthcare providers can scale medical imaging processes to meet growing demands. Besides, automated standard tasks free radiologists for more complex cases. It also enables remote interpretation of images, giving more people access to specialist expertise.
More accurate image interpretation
AI is trained to extract and analyze geospatial data to identify intricate or hidden patterns within imaging data that often escape human eyes. In addition to that, AI can be used to improve image clarity and detail for more accurate analysis.
When integrated with medical systems, it provides exceptional capabilities to accurately interpret X-ray, CT, MRI, and other imaging results.
For example, a recent study found that CNN-aided computer systems can detect brain tumors in MRI images with up to 98.56% accuracy.
Accessibility of diagnostics in a variety of clinical settings
AI-assisted medical imaging enables clinicians to analyze and process imaging data beyond medical departments or facilities. Patients can receive imaging reports on mobile apps integrated with imaging systems. Likewise, clinics in rural areas facing limited resources can use portable AI diagnostic tools.
More effective and cheaper treatment
AI allows hospitals to optimize their resources and healthcare workforce for better patient outcomes. Instead of spending substantial time on screening and analyzing the results, clinicians use AI-generated summaries to scale patient treatment. Medical image AI solutions also help healthcare providers reduce expenses by eliminating repetitive and redundant processes.
Common types of AI Computer Vision applications in Healthcare
From pre-screening to long-term treatment, AI imaging solutions offer wide-ranging possibilities for radiology use cases. While this list is far from exhaustive, I did my best to highlight the core examples of AI use cases in medical imaging analysis.
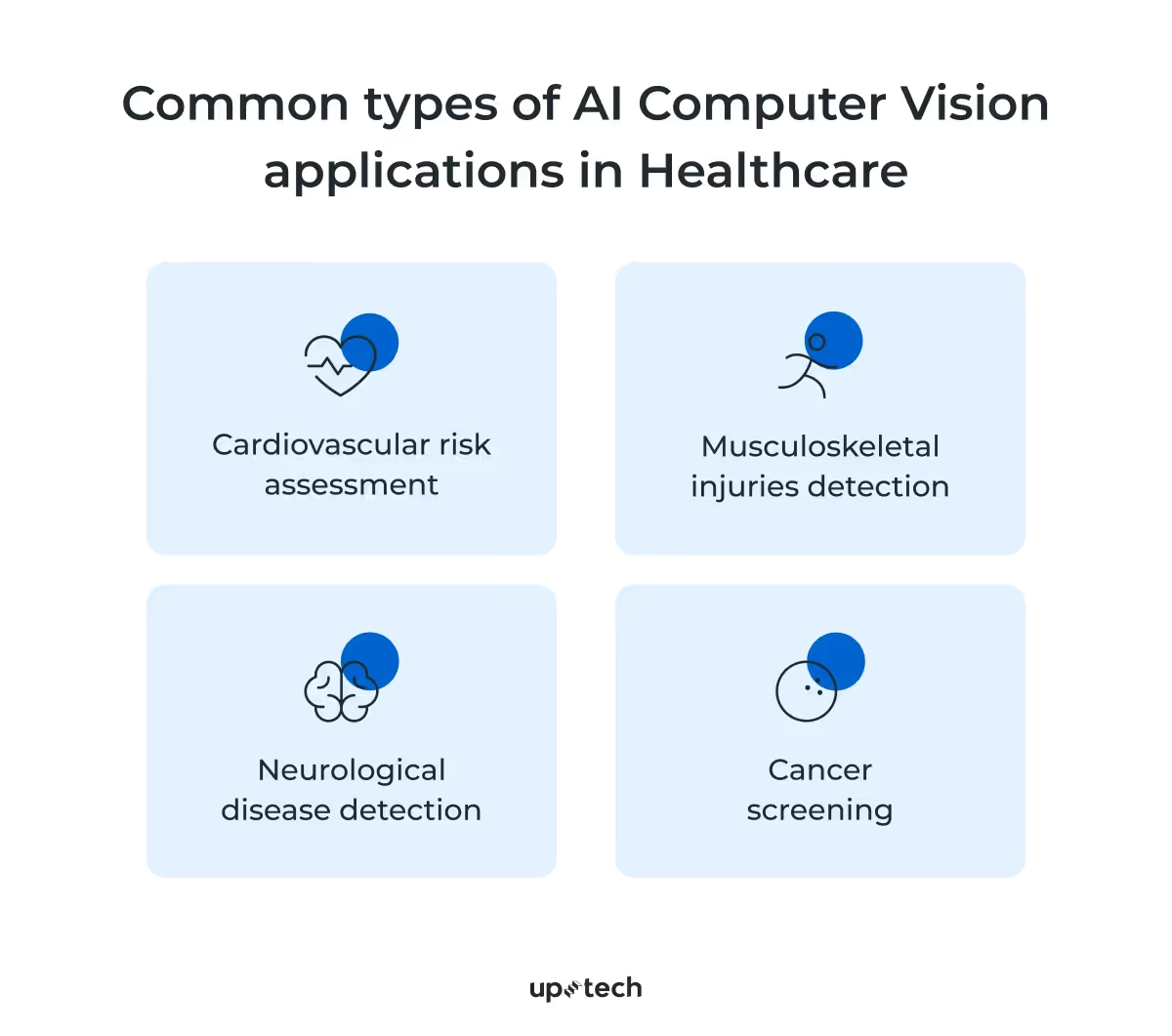
Cardiovascular risk assessment
AI diagnostic tools can augment visual inspections in cases of symptomatic complaints of cardiovascular diseases. During preliminary inspections, radiologists obtain reports from x-rays and radiographs, which they can feed to the AI tool for further analysis. Deep learning models allow physicians to narrow down the symptoms, such as artery blockages, and render appropriate treatments.
Musculoskeletal injuries detection
Imaging systems are instrumental in assessing musculoskeletal injuries. However, some fractures, tissue injuries, and abnormal musculoskeletal conditions might be overlooked in trauma assessments. With AI, physicians can be alerted of the subtle signs picked up by initial radiology scans. Studies have observed that analyzing CT scans with CNNs will likely improve clinical evaluations of fractures.
Neurological disease detection
Brain studies for detecting neurological diseases are challenging with existing medical imaging technologies. Often, it involves iterative segmentation, comparison, and assessments of possible biomarkers detected in the brain MRI. Using AI for diagnostics improves accuracy and speed in classifying possible diseases while reducing false positives.
Cancer screening
Medical imaging is instrumental in detecting tumors and precursors of common cancers. The challenge that healthcare providers face is to ascertain possible malignancies accurately. AI helps physicians diagnose and provide appropriate treatment by supporting cancer prognosis. It prevents unnecessary invasive treatment in false positive cases and ensures early detection of cancerous cells.
How to Use AI for Medical Image Analysis
At Uptech, I and my team have implemented AI features in new and existing products. We follow specific steps to balance cost, model accuracy, and time-to-market.
In this section, I’d like to focus on medical image analysis using AI in more detail. I’ll guide you through the key steps required to build a custom software solution for it to function as an AI medical report analyzer.
The main steps for building an AI-powered medical imaging platform are as follows:
- Define the purpose of your medical imaging AI platform
- Collect and prepare training datasets
- Choose the right AI tech stack for medical image analysis
- Train the ML-model
- Integrate and test your AI medical report analyzer
- Launch your AI-powered medical imaging platform
- Monitor and upgrade software

Below, I will explain each step in detail.
1. Define the purpose of your medical imaging AI platform
The use of AI separates medical image analysis platforms from conventional radiology machines, such as X-ray and CT scanners. With AI, data scientists can train the medical imaging tool to recognize specific disease representations with annotated datasets of medical images.
In simpler words, the medical image AI model learns to predict what a disease looks like after learning from various images of the disease.
So before you jump into the AI ocean, make sure you need its power in the first place. There’s no need to get AI power just because it’s trendy.
First and foremost, you must determine the goal and problems your product will solve in the healthcare space and specific areas where artificial intelligence can assist.
One case is an AI tool designed for breast cancer screening. Such a tool can detect subtle microcalcifications in mammograms that might be too small for a radiologist to notice. This capability leads to earlier detection and treatment and, as a result, significantly improves patient outcomes.
Other AI systems might focus on automating the classification of various lung conditions, such as distinguishing between pneumonia, tuberculosis, and COVID-19 in chest X-rays.
These are two completely different problems (outlier detection and classification, respectively) that require different approaches and, therefore, technology stacks.
2. Collect and prepare training datasets
Based on the project goal set at the first stage, you move to the next phase – training data collection and preparation.
Here’s when all the fun begins, as getting enough quality medical data is a real quest. Of course, you need to focus your effort on curating medical datasets related to the product’s purpose. If you’re building an AI tool for cancer detection, you’ll need images with different variations and stages of malignancies. A diverse, equal representation of the subject ensures a fairer imaging model.
But this is half the battle.
All computer vision tasks (developing an AI medical report analyzer is no exception) require a lot of high-quality training data. The more diverse in geography, gender, age, and race, the better. But where do you get it?
There are 2 possible options:
1. Processing existing data
You may already have some medical image data in place. However, it still needs to be processed to comply with all the standards and regulations.
Preparation activities include but are not limited to the following ones:
- Data anonymization. DICOM saves protected health information (PHI) such as name, age, date of birth, ID number, etc. Such data must be anonymized by being removed from the images to comply with regulations like HIPAA and GDPR. This can be done using tools like DICOM Anonymizer or by converting DICOM files into non-DICOM formats like PNG or JPEG, which are easier to handle.
- Data labeling. Since medical images contain information that only certain specialists can read and understand, it’s important to engage medical experts to annotate the images. They mark and outline specific areas in the image, such as an organ, nodule, tumor, or lesion. This way, you create a ground-truth dataset necessary for training AI models. This step can be resource-intensive, often requiring multiple experts to ensure accuracy.
2. Using public datasets
If you don’t have private datasets, public datasets can be a good alternative. While they come with limitations, such as less control over data diversity and quality, they provide a quick and cost-effective way to gather data.
Public datasets can be found on websites like Kaggle, and they are widely used for AI development:
- NIH Chest X-rays. This dataset contains over 112,000 de-identified radiographs for lung disease classification, annotated with NLP to extract abnormality data.
- SIIM-ACR Pneumothorax Segmentation. A dataset for identifying pneumothorax in chest X-rays.
- Chest Xray Masks and Labels. This dataset is prepared for lung segmentation tasks.
The abovementioned public datasets can be invaluable for small to medium-sized businesses and researchers. They enable the development of MVP (minimum viable product), prototypes, and PoC (proof-of-concept) tools without the hefty data collection and labeling costs.
3. Choose the right AI tech stack for medical image analysis
Compare imaging models’ performance against machine learning benchmarks and choose the one that works best in the specific use case.
For example, several models have been tested against the retina, fracture, adrenal, and other MedMNIST datasets. If you’re diagnosing pneumonia, Google AutoML Vision proved the most accurate.
Speaking of deep learning AI for medical image analysis, here are the most commonly used models.
CNNs
Many imaging models are variants of convolutional neural networks (CNN) – the most efficient model for this task and a key block in the configuration of deep networks.
CNN consists of multiple hidden convolutional layers that allow it to extract geospatial data efficiently from image sources with minimal preprocessing. A very deep network can have over 100 layers, but these layers are typically categorized into three main types:
- Convolutional (conv) layers. These are the core of CNNs. Filters called convolutional kernels scan the image, pixel by pixel, to detect patterns. Early layers identify basic shapes, like the edges of the organ. Later layers detect more complex structures, such as nodes or abnormalities. The results are called feature maps.
- Pooling layers. These layers follow conv layers to reduce the size of feature maps, making the data easier to process and requiring less computational power.
- Fully connected (FC) layers. These layers take all the information from previous layers to make the final decision, like classifying what the image shows.
In essence, CNNs work as a two-part system: the first part extracts features from the image, and the second part classifies the image based on those features.
There are many CNN variants capable of detecting and classifying objects in radiology images. Examples of these are LeNet, AlexNet, ZFNet, GoogleNet, VG-GNet, and ResNet.
Transformers
Transformers, first described in a 2017 paper from Google and originally developed for understanding sequential data in NLP, are the type of AI that can also be used for medical image analysis.
These models can identify complex relationships within data by using attention mechanisms, which allow them to focus on important parts of the input data.
In medical image analysis, transformers process and analyze images by capturing spatial relationships and patterns, similar to how they understand context in text.
This makes them effective for tasks like
- image segmentation, e.g., identification of the boundaries of organs or abnormalities
- image classification, e.g., determining the presence of diseases from imaging data.
Transformers work through two main components: the encoder and the decoder. The encoder extracts features from the image, while the decoder uses these features to produce the final output, such as a labeled image or a classification decision. The attention mechanism helps them focus on key areas of the image, improving the accuracy of the analysis. Additionally, transformers can process multiple images all at the same time.
An interesting imaging model based on the transformer architecture is the Segment Anything Model (SAM) by Meta AI. SAM can identify and create a boundary on any objects from prompts with zero-shot learning. Researchers have adopted SAM for medical imaging by enabling the model to process DICOM images in a study. They found the model generalizes well even on complex pathology images.
Generative Adversarial Networks (GANs)
Generative Adversarial Networks (GANs) are another type of advanced deep learning algorithm used in the development of medical image analysis platforms.
Inspired by game theory, the GAN algorithm consists of two competing neural networks:
- the generator that creates fake samples
- the discriminator that tries to distinguish between real and fake samples.
The generator starts with random inputs to produce fake data, and the discriminator acts as a judge to determine if the data is real or fake. During training, the discriminator learns from labeled real and fake images, with a feedback loop being created that enhances both networks' abilities.
The ultimate goal is for the generator to create images so realistic that even humans can't tell them apart from real ones. The discriminator, in its turn, continues to get better with its judgment.
Of course, this list of deep learning AI models for medical image analysis isn’t exhaustive, and there are other variants to consider.
4. Train the ML-model
While it's possible to train your own AI model from scratch, we do not recommend it due to the immense computational resources needed. Instead, a more efficient approach is to fine-tune pre-trained models with the medical datasets you’ve prepared.
What does that mean?
Basically, you take a model that has already been trained on a large, general dataset and refine – fine-tune – it with your specific medical images. Pre-trained models like ResNet, VGG, or ViT (Vision Transformers) have already learned to recognize a wide range of patterns and features in medical images.
So, what you do is adjust the hyperparameters until the model converges to the required inference level and its performance meets your specific needs.
Parameters in an AI model are the elements that the model adjusts during training to learn from the data. For example, in an image analysis model, parameters help the model identify features like edges, shapes, or colors.
Hyperparameters, on the other hand, are settings that you configure before the training begins, such as the learning rate or the number of layers in the model. Adjusting hyperparameters controls the model's learning and can significantly impact its performance and accuracy.
Training a medical imaging AI tool requires collaborative efforts from machine learning engineers and medical experts. An AI computer vision application must be trained with various pathological representations to infer with strong confidence, even for marginal cases. This is critical to ensure physicians are supported with accurate results when diagnosing and treating patients.
5. Integrate and test your AI medical report analyzer
After training your AI model, the next step is integration. Set up a seamless data pipeline between the product and the AI model by using APIs or other means of communication. Also, remember to secure all data points from adversarial risks.
Once integrated, the AI medical imaging tool will be put into several test stages. You would typically evaluate the model for:
- Bias. The model must not favor or disadvantage any group based on attributes like age, gender, or race.
- Accuracy. This metric checks how often the AI correctly identifies and classifies medical conditions.
- Overfitting. This one tests whether the model performs well on new, unseen data. Overfitting occurs when a model learns the training data too well, including noise and outliers, which can reduce its ability to generalize to new cases.
Other metrics may include sensitivity, specificity, and precision, among others.
You’ll need to refine the model with additional fine-tuning if it fails to generalize on actual image data. This is done either through adjusting the model's parameters or using more diverse datasets to improve its ability to generalize across different types of medical images.
Finally, conduct real-world testing in a controlled environment before full deployment. This helps identify any unforeseen issues and ensures that the AI tool integrates smoothly into clinical workflows.
6. Launch your AI-powered medical imaging platform
Now, your AI medical imaging tool is ready for public use. Start by launching the product to a specific department, such as radiology or oncology, where you can closely monitor its performance and gather initial feedback.
When the tool has proven stable, you can scale it facility-wide or plan a phased expansion to other departments. This scaling should include comprehensive training for all staff members so that they can effectively use the new technology.
In the meantime, be prepared to handle post-release bugs and technical issues. The perfect-case scenario is to have a dedicated support team to address any problems that arise quickly. Also, collect feedback from users to identify potential improvements and new features that could enhance the tool's value.
7. Monitor and upgrade software
Both AI and medical disciplines undergo rapid changes, and so should your product. Observe the model to ensure it performs consistently. Maintain a focus on continuous improvement.
Regularly update the platform with new features and advancements in AI and medical imaging to meet changing regulations, optimize model performance, improve data accessibility, and more when necessary.
Using artificial intelligence for medical image analysis means that you have to keep your finger on the pulse of all the changes going on.
5 Commercially Available Tools That Use AI in Medical Imaging and Diagnostics
Wanting to make the most of deep learning AI for medical image analysis doesn’t necessarily mean building such a software solution yourself (though custom AI medical report analyzers always win in this battle). Nevertheless, I have rounded up a few commercially available solutions for you to consider.

Please note: I don’t promote any of the solutions presented. They are listed exclusively for your reference.
- ClariCT.AI by ClariPi is a South Korea-developed and FDA-cleared tool that helps improve the clarity of low-dose and ultra-low-dose CT scans by reducing noise. In this way, the tool allows radiologists to be more confident in their readings.
Behind ClariCT.AI, there’s the CNN model that was trained with over a million images, focusing particularly on lung cancer screening.
- Breast Health Solutions by iCAD is the AI suite that uses deep learning to analyze 2D and 3D mammograms and assess breast density. One part of this suite, called ProFound AI, was the first AI technology approved by the FDA for 3D mammography.
- KardiaMobile by AliveCor is a personal ECG device that captures an ECG in 30 seconds. The mobile app also uses AI to detect slow and fast heart rhythms, atrial fibrillation (AF), and normal rhythms. Users can send these ECG recordings to their clinicians for further review.
- SkinVision is an AI-powered app that helps users assess the risk of skin cancer from photos of suspicious moles or marks. Its AI algorithm, trained on 3.5 million images, has helped diagnose 40,000 cases of skin cancer. While the app is available worldwide, except in the US and Canada, it should not replace a visit to a dermatologist.
- IDx-DR is the first AI system approved by the FDA for diagnosing diabetic retinopathy (DR). It works exclusively with the Topcon retinal camera and provides one of two recommendations: seeing an ophthalmologist for more severe cases or scheduling a rescreen in 12 months for milder or negative results.
While these off-the-shelf medical imaging AI platforms offer valuable capabilities, they come with limitations. They may not fully meet your specific needs and lack the flexibility and customization that a bespoke solution can provide.
Challenges of Using AI in Medical Image Analysis and Ways to Tackle Them
As promising as AI medical diagnosis sounds, there are several concerns that founders and developers face. After all, AI is still at its infant stage, with new findings influencing development efforts. Moreover, the medical industry is highly regulated, presenting several factors that need a deeper look.
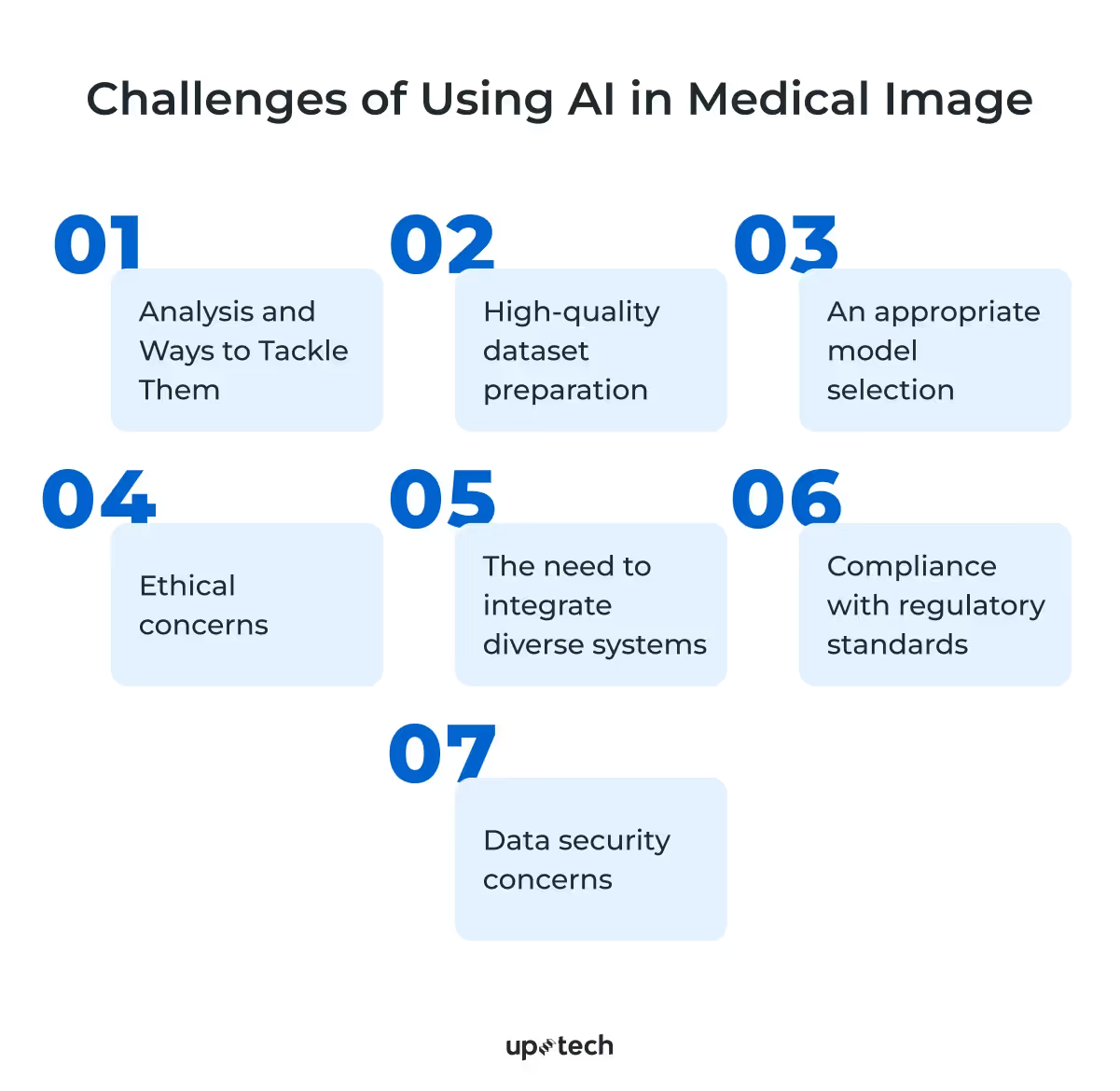
So, here are the main issues you may face when using artificial intelligence for medical image analysis and tips for handling them effectively.
High-quality dataset preparation
Medical imaging AI uses domain-specific datasets to train the underlying deep learning model. The dataset contains annotated laboratory images that enable the model to learn what specific symptoms look like. Any negligence in preparing the dataset will directly impact the imaging model, leading to inaccurate or biased predictions.
When training the model, curate high-quality datasets. This means that you must use datasets that fairly represent the pathological symptoms and patients to minimize bias and ensure model accuracy. Models trained with questionable datasets may produce erroneous results and negatively impact patient outcomes.
An appropriate model selection
Convolutional neural networks and transformers are widely used for image detection and classification. However, each variant model has comparatively different performance. For example, some might be more prone to hallucinations (when an AI model mistakenly detects patterns or features that aren't actually present in the medical images) than others.
So, it’s super important to evaluate the model of choice for key inference metrics before choosing a model for medical imaging.
Ethical concerns
Medical imaging models inherit bias and inaccuracies from their training dataset. When applied to patients, they may produce incorrect diagnoses, which physicians must resolve. Besides, regulatory bodies might express concerns about how companies curate medical datasets to train the AI model.
To address ethical concerns, you must enforce data governance that will serve as a solid foundation for a fair and inclusive medical imaging AI platform.
In addition to that, some pre-trained models might exhibit harmful or abusive behavior when interacting with users. Never deploy them as they are. Instead, fine-tune them with methods like reinforcement learning with human feedback (RLHF) to ensure they are helpful and can follow instructions.

The need to integrate diverse systems
Medical imaging AI needs access to radiology results generated by existing machines. To do that, you must ensure disparate systems across medical facilities can exchange data reliably with the AI platform. This involves standardizing data formats like DICOM or upgrading their networking capabilities.
Compliance with regulatory standards
Healthcare providers must comply with applicable regulations or risk hefty penalties when introducing new apps or equipment. For example, hospitals in the US are subjected to HIPAA, which specifies guides for protecting patients’ health data. Meanwhile, AI imaging systems targeting the European market must adhere to the General Data Protection Regulation (GPDR).
Keep in mind that most providers, including OpenAI, do not allow their models to be used for medical use cases. You must sign a business associate agreement with the provider to use its AI models for HIPAA workloads. Therefore, deploying open-source CNN or computer vision transformer models on a secure cloud infrastructure is better. It allows you better control of data governance, policies, and security according to HIPAA guidelines.
Data security concerns
AI applications move vast amounts of data across connected storage, increasing data risks. Rising data breaches affecting medical facilities, as reported by ENISA, called for encryptions, access management, and other measures to produce digitally resilient medical AI.
Hence, those interested in developing AI medical report analyzers must apply security best practices in the AI system and cloud infrastructure to secure patients' data and comply with HIPAA. This involves encrypting the database, applying multi-factor authentication on endpoints, and other security measures.
Read our dedicated article to learn more about app security best practices.
To sum it up, you must also remember that AI is not and will not be a substitute for human expertise; radiologists and other healthcare professionals are still needed to interpret AI-generated findings and make clinical decisions.
How can Uptech help?
Uptech helps international companies build digital AI products that solve real problems. Our multidisciplinary team comprises AI experts who augment developers, designers, and other IT specialists. We’re constantly equipping ourselves with the latest machine-learning knowledge and applying it to our projects.
For example, we built Hamlet, an app that uses a language model to generate summaries from text or PDF documents. We integrated the text-DaVinci-003 model with the user interface and backend infrastructure to provide our US client with a scalable AI text summarizer.
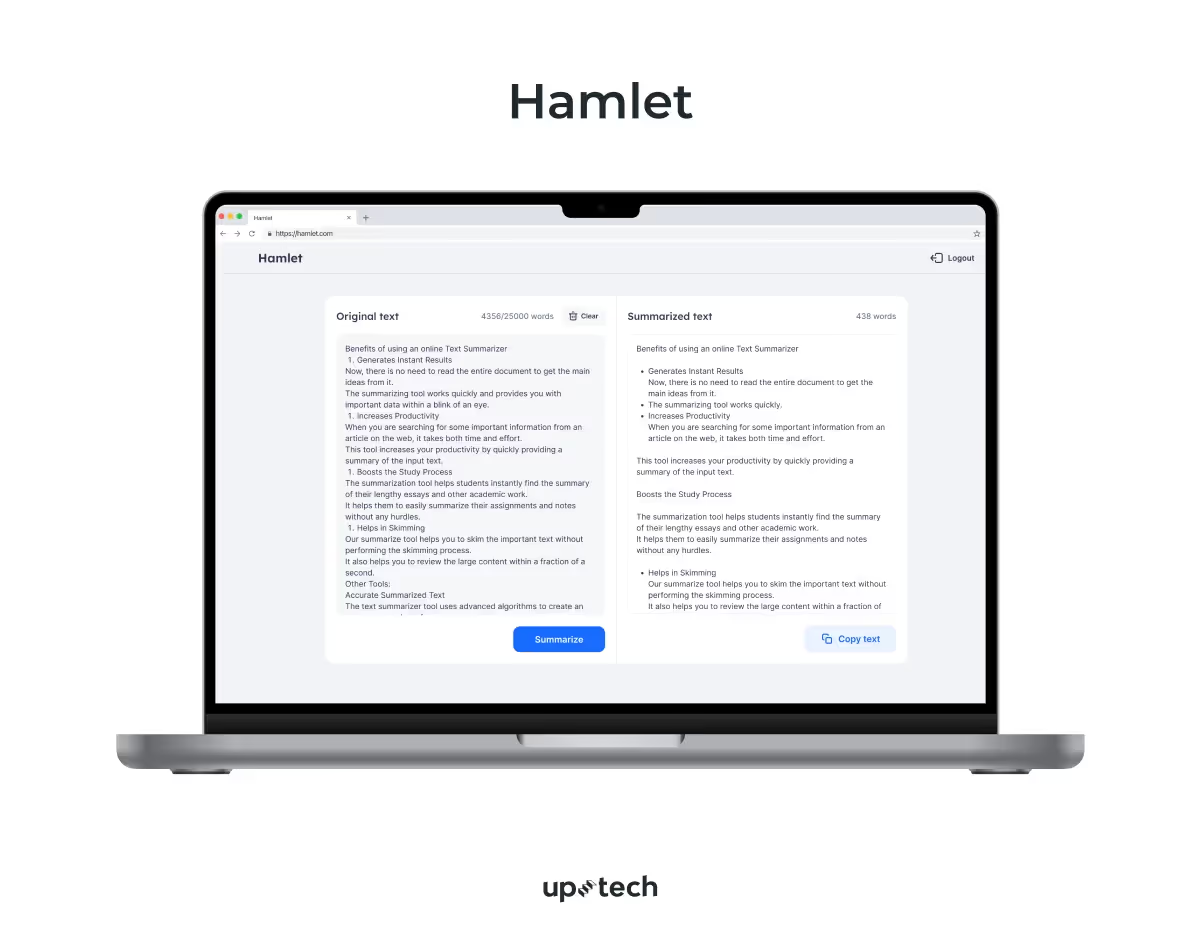
Our experience also extends to the healthcare industry, which faces demanding compliance and security challenges. In this project, we built a mental healthcare app enabling users to interact with trained therapists on a mobile app. We thoroughly surveyed users and the healthcare marketplace to provide value-added differentiations such as in-app chat, calendar, and learning modules.
At Uptech, we’re sensitive to your concerns about cost, timeline, and expertise. Our stellar portfolio indicated that we’re consistent with deliverables. Besides that, we can also meet tight deadlines and budget requirements. For example, our team can deliver a proof of concept (PoC) in 1 month for $20K.
Talk to our team to learn how we did it and to learn more about AI development for medical imaging.