As healthcare institutions scale, they struggle to turn increasingly growing volumes of medical records to their advantage. Doctors, nurses, insurance agents, and medical staffs rely on medical record summaries to make informed decisions and support patients throughout their healthcare journey. Recent advancement in deep learning technologies makes AI medical record summarization an attractive solution for healthcare providers.
The emergence of generative AI technologies primed AI for significant growth in the healthcare industry. According to Statista, the global AI healthcare market will be worth $188 billion by 2030, a 37% increase from its value in 2022. Therefore, building AI-powered solutions, such as medical summarization tools, is a smart move for startups, healthcare institutions, and product managers.
As the co-founder and tech leader at Uptech, I’ve closely followed the impact of AI in various industries, including healthcare. We’ve also been testing the practical applications of generative AI technologies by building or integrating genAI with clients’ products. For example, we built Hamlet, an app using generative neural networks to summarizes PDFs and text documents.
In this article, I’ll share how using AI will bring efficiency to medical records summarization. I’ll also explore the challenges and best practices when implementing AI summarization on medical records.
What is Summarization of Medical Records
Medical records contain the historical prognosis, treatment, lab reports, and other medical notes critical to the patient’s medical interest. In conventional practice, doctors, nurses, and medical staffs take on the administrative tasks to analyze, organize and fill in the missing gap in the patient’s treatment records.
Summarized medical records benefit several parties when rendering services to patients.
- Medical summaries for law firms enable patients to pursue litigation options with a stronger legal foundation. The summaries detailed their medical histories, costs, treatment, and other relevant information that aid their case.
- Insurance firms use medical summaries to assess claims and reimburse patients fairly. In this case, an AI-generated summary provides clear, concise, and objective representations to support the patient’s claim.
- Medical summaries allow healthcare providers to consolidate siloed patients’ health information in different departments. Medical summarization improves the overall visibility of the patient’s information, making doctors more accurate when rendering prognosis or treatment.
While healthcare providers have strong motivations to summarize patients' data, the process is not straightforward. Medical data are presented in several formats, each regularized by their respective standards.
- C-CDA, an acronym for Consolidated Clinical Document Architecture, is a popular format that US healthcare providers use to exchange patient information. C-CDA stores healthcare data in XML, providing a patient’s medical history timeline.
- FHIR (Fast Healthcare Interoperability Resources) is a data-sharing standard that promotes clinical interoperability between and amongst medical institutions. It specifies APIs that enable different apps to share medical data reliably across departments.
- HL7 (Health Level 7) is a framework that supports EHR sharing between healthcare providers. It provides messaging formats and protocols that improve efficiency and accuracy for care deliveries.
- SNOMED CT is a comprehensive medical terminology that healthcare providers use to automate healthcare data processing. It allows healthcare systems to maintain consistent definitions, concepts, and entity relationships throughout the data-sharing pipeline.
- ICD (International Classification of Diseases) is a global standard for documenting diseases, injuries, causes of death, and other mortality data with specific codes.
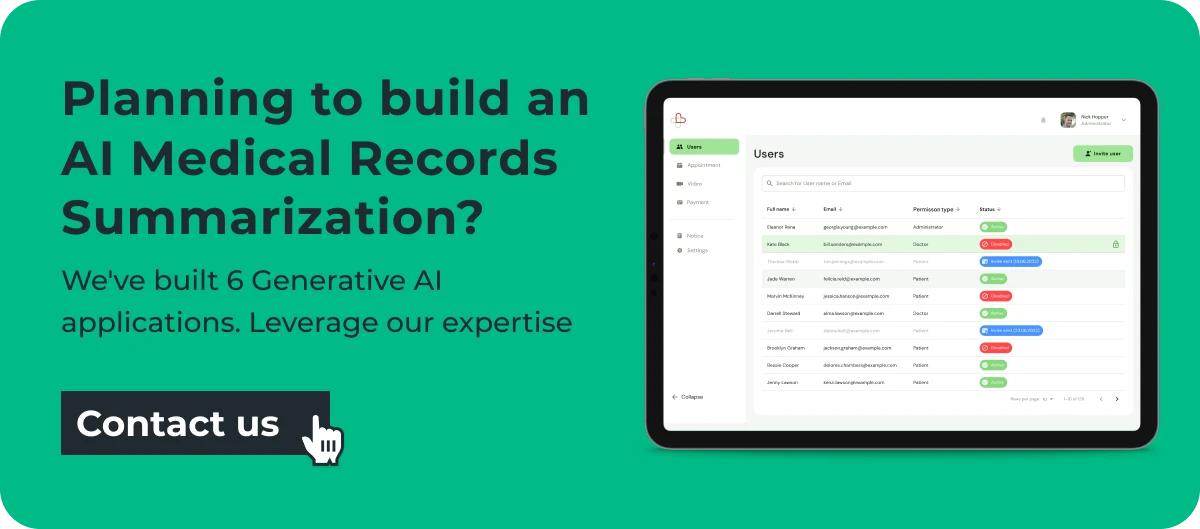
Why Should Health Providers Summarize Medical Documents
Medical records in their original form are extensive and useful for specific medical interventions or legal claims. However, most of these data are confined to different systems, which limits their potential to drive data-driven decisions. Summarizing the vast numbers of medical data streamlines the healthcare system in different ways.
Improve patient care delivery
Healthcare professionals can better render the appropriate treatment without spending valuable time sorting and searching for patients’ records. With readily-available medical summaries, they can perform administrative tasks, such as finalizing lab reports, more efficiently.
Consolidate disparate medical data
Medical summarization expands the value of health data collected and stored by different departments. By extracting key information from various medical sources, healthcare providers can summarize patients’ health conditions and monitor their progress without repetitive manual intervention.
Enable virtual assistant
Summarized patient data also allows healthcare providers to train and deploy intelligent virtual assistants. The virtual assistant can learn from the extracted information and personalize patient interactions.
Supports medical research and education
Summarizing medical records build up a condensed and organized online knowledgebase for medical researchers and students to further their learning. It speeds up education by making key facts easily accessible to learners.
Critical Issues of Summarizing Medical Documents
Summarizing medical documents benefit healthcare providers, but several factors complicate such efforts.
Comprehending Biomedical Text
Medical data consists of complex terminologies specific to the healthcare industry. It takes a medically accredited professional, such as a physician, therapist, or pharmacist, to extract and summarize medical text without losing its original meaning.
Extracting relevant information
Besides complexities, medical records hold detailed information dictating prognosis, treatment, lab results, and other data specific to a patient’s condition. When summarizing, extracting information specific to the required task is essential, which proves challenging for untrained individuals.
Producing comprehensive summary
While medical summaries compress patients’ medical history, they must detail all the important records. For example, you’ll need to extract information from admission notes, consent for treatment, lab results, surgery reports, and prescription lists to provide a concise summary.
Dealing with AI hallucination
If you use generative AI to assist in summarizing medical records, you must be mindful that AI is prone to hallucinations. In simpler words, the AI might make up an incorrect but believable narrative from the extracted information.
Analyzing patterns and trends
Manual record summarization is tedious and might not be practical for spotting specific patterns or potential correlations in the data. Humans have limited capacities for gathering and processing vast information presented in the records.
Human errors
The summaries' accuracy depends on the medical or administrative officers who prepare them. As humans, they might not be consistent in ensuring the summary’s correctness and completeness.

How AI Medical Documents Summarization Benefits Medical Affairs
Medical Affairs are responsible for propagating medical information amongst clinical practitioners, patients, healthcare providers, and other entities in the industry. This includes generating summaries of medical literature, patient data, and other healthcare documents. Manual summarization cost Medical Affairs time, expenses, and missed opportunities to support patients and medical providers.
In this sense, generative AI is a potentially transformational technology that benefits the Medical Affairs team greatly. According to McKinsey, using Gen-AI to analyze clinical health records can unlock $1 trillion of opportunities. You wouldn’t want to miss the opportunity that AI presents in advancing medical summarization workflows.
Here’s why.
1. Reduce review time
Medical researchers and officers spend several minutes reviewing a page of medical records. With Generative AI, they save up to 90% of their time while accurately extracting essential information.
2. Accelerate decision-making
Medical Affairs oversee various activities, including clinical trials, creating medical strategies, and disseminating accurate medical information. They can use AI-generated medical records to support critical decisions affecting the outcomes of such activities.
3. Improve accessibility
With generative AI, Medical Affairs can translate medical content into multiple languages with less effort. This provides the global medical community equal access to medical literature. Moreover, AI can reproduce such content with languages understandable by non-technical audiences.
4. Detect errors
Generative AI can quickly compare medical records with established ground truth data to spot errors. This prevents erroneous data from being put into circulation, which may affect public safety and patient well-being.
5. Identify patterns and anomalies
Deep learning models that generative AI uses consist of artificial neural networks capable of analyzing complex patterns. Medical teams can use AI to detect anomalies that med workers might overlook in manual reviews.
6. Save cost
Summarizing AI records help healthcare companies reduce substantial expenses, including physical storage, logistics, and the workforce involved. The Medical Affairs team can also optimize and reallocate their resources to higher-value tasks.
7. Reduce carbon footprint
Medical companies pursuing environmentally sustainable goals will also benefit from medical records summary services. Instead of storing volumes of paper records, the move shifts their medical knowledge to private cloud storage.
8. Improve work-life balance
Medical workers burdened with manual summarization tasks will enjoy a welcomed respite when integrating medical summarization with AI. They can divert their effort to supervisory roles and deliver more to the healthcare ecosystem.
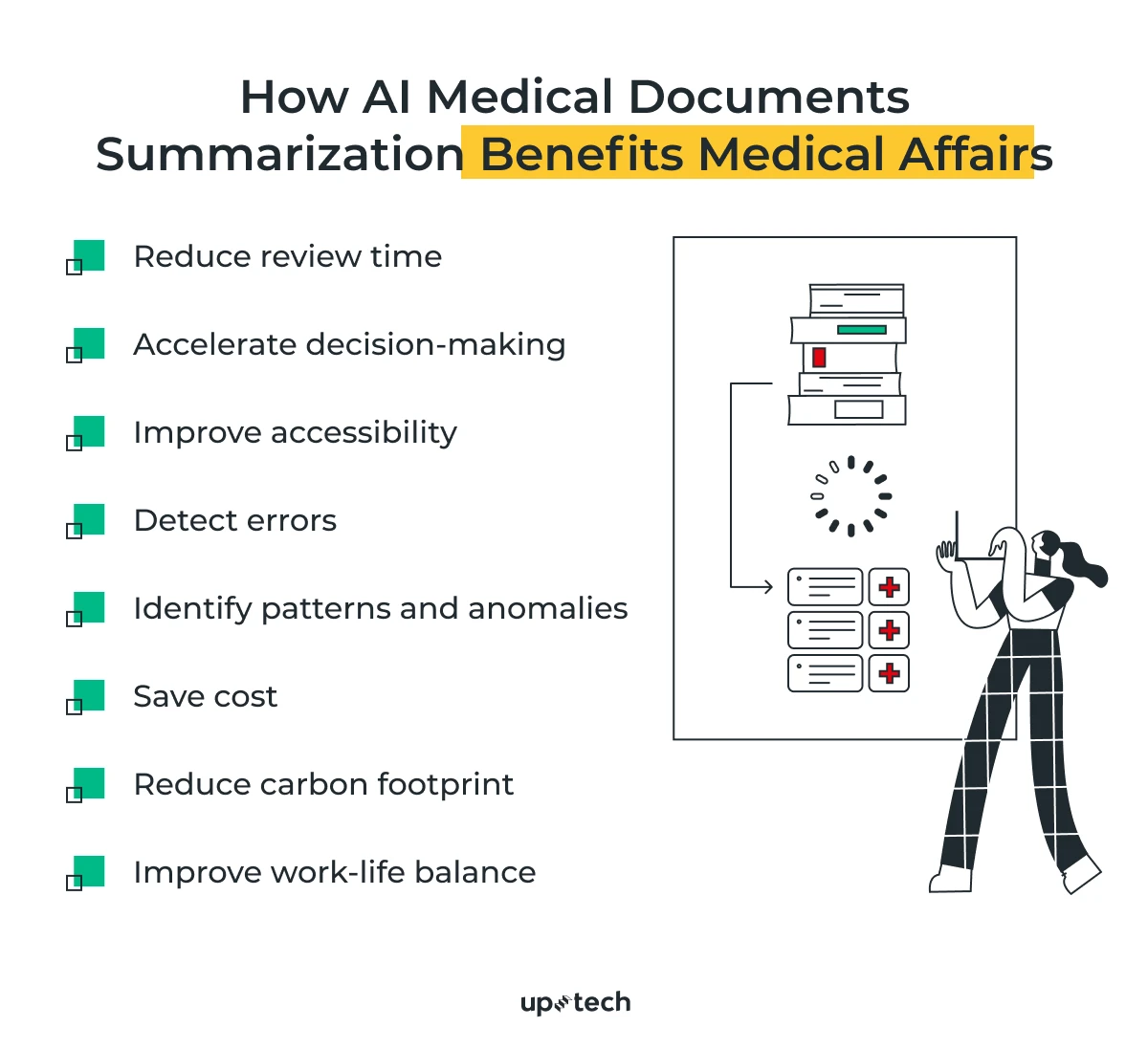
Challenges of Developing AI-driven Summarizing Medical Documents
Despite AI's overwhelming benefits, there are concerns when applying AI-powered medical summarization at scale. Moreover, the medical industry is tightly regulated and requires strict compliance by all stakeholders. So, pay attention to these challenges when building AI summarization tools.
Preparing quality training datasets
You must train the underlying deep learning model with high-quality datasets to produce accurate AI summarization tools. Otherwise, the resulting machine-learning model might suffer inaccuracy, bias, underfitting, and other performance issues. Compiling quality datasets for medical AI requires curating and annotating diverse types of sources, including lab results, personal health data, and administrative notes. You might have to commit substantial resources and time to preparing the datasets.
Choosing the right foundational model
AI summarization apps use NLP and computer vision models to extract data from unstructured resources. There are many such models available from private ML vendors and open-source communities. However, it’s crucial to choose the right model by assessing their performance on existing benchmarks. For example, a language model’s truthfulness score might indicate its likelihood of hallucinating when summarizing unseen data.
Navigating ethical challenges
Generative AI models lack explainability and transparency, which triggers concerns about accuracy, security, and bias when summarizing health records. Healthcare providers must be aware of such risks affecting patient trust and outcomes when medical staffs rely on AI-generated summaries to make decisions.
Integrating with existing medical systems
When deployed, the AI summarizing app must be able to extract data from existing medical systems. Enabling interoperability amongst disparate medical systems is challenging, as each may function with its own standards and protocols. Moreover, some healthcare providers still operate with legacy paper-based systems, which require the AI summarizer to be fitted with optical character recognition (OCR) technology.
Mitigating the lack of standardization
Medical facilities are governed by differing healthcare regulations specific to their countries or regions. When expanding your AI solution to different regions, you must comply with the necessary acts. For example, US health providers must comply with HIPAA and HITECH Act when implementing IT solutions. Meanwhile, healthcare frameworks like EHDS, GPDR, and NIS 2 regulate medical technologies in European countries.
Ensuring data security and privacy
Given that AI summarizers process large numbers of sensitive health data, you must ensure their data pipeline is resilient against adversarial threats. Implementing data security measures like encryption and access authorization are mandatory to safeguard patients’ privacy. However, securing numerous data exchange points might prove daunting for AI development teams.
Assessing legal implications
Besides technical complications, developers face legal risks when applying AI summarizers to healthcare facilities. Medical professionals use the AI app to generate accurate summaries, which they later use to diagnose, treat and support patient recoveries. Any inaccuracies affecting the AI solution could severely impact patient outcomes.
.webp)
HIPAA Compliance Concerns when Using Gen AI for Summarization
One of the most straightforward ways to build a medical record AI summarizer is to leverage an existing pre-trained deep learning model. For example, developers can use OpenAI’s APIs to access ChatGPT's functionality. Unfortunately, OpenAI and many genAI providers prohibit using their models for HIPAA-regulated use cases as of 2023. So, this rules out using publicly-available models like ChatGPT unless you can strike up special contractual agreements for HIPAA-enabled data protection.
Alternatively, you can deploy other open-source large language models (like LLaMA, PaLM, etc) on cloud servers. Doing so gives you greater control and transparency when securing the data the model uses. For example, developers can use HIPAA-aligned services on AWS to store, process, and analyze protected health information.
Even with secure data and cloud infrastructure, developing a HIPAA-compliant summarizer requires coding practices aligned with the policy. For example, your app must automatically log out all users and back up all data.
Learn more about developing HIPAA-compliant software here.
Choosing AI Tech Stack for Summarizing Medical Documents
The emergence of deep learning models provides more technological options when building AI summarizers. However, not all summarizing apps need state-of-the-art language models. Depending on their use case, you can create certain AI apps with simpler machine learning algorithms.
I share several types of machine learning models that could form the building blocks of your app.
Deep learning AI models
Deep learning models are neural networks with multiple hidden layers capable of efficiently extracting and analyzing vast information. Large language models like GPT-4, Bard, and LlaMa are exceptional in processing textual information. Meanwhile, generative AI like MidJourney and Dall-E are engineered to understand visual data.
Neural networks
Basic machine learning models like convolutional neural networks (CNN), recurrent neural networks (RNN), and Bayesian networks can be adapted for NLP tasks, including text mining and establishing semantic relationships. They are also less resource-intensive to train, making them preferable options for some AI apps.
Image classification models
Models like VGG-16, ResNet50, and Inceptionv3 are variants of convolutional neural networks. CNN uses convolutional layers to extract the finer details in graphical data effectively. Such models enable developers to build AI summarizers capable of segmenting, analyzing, and classifying medical images.
When choosing AI models, consider their training time, performance, and compliance. Deep learning models take significantly longer time and more computational power to train than simpler algorithms. In most cases, it’s more practical to fine-tune a pre-trained model than training a foundational model from scratch.
As mentioned, most model providers do not support use cases in healthcare systems. Neither do all models offer similar performance. So, be diligent and choose a model that excels in areas your app requires. Then, train and deploy the model in a secure infrastructure and connect it to a regulatory-compliant app.
8 Steps to Build an AI Medical Records Summarizer
Generative AI is an emerging discipline that many software teams strive to adopt into their practice. At Uptech, we use a framework for developing AI apps that solve users’ problems. When building AI summarization apps, here’s what you should do.
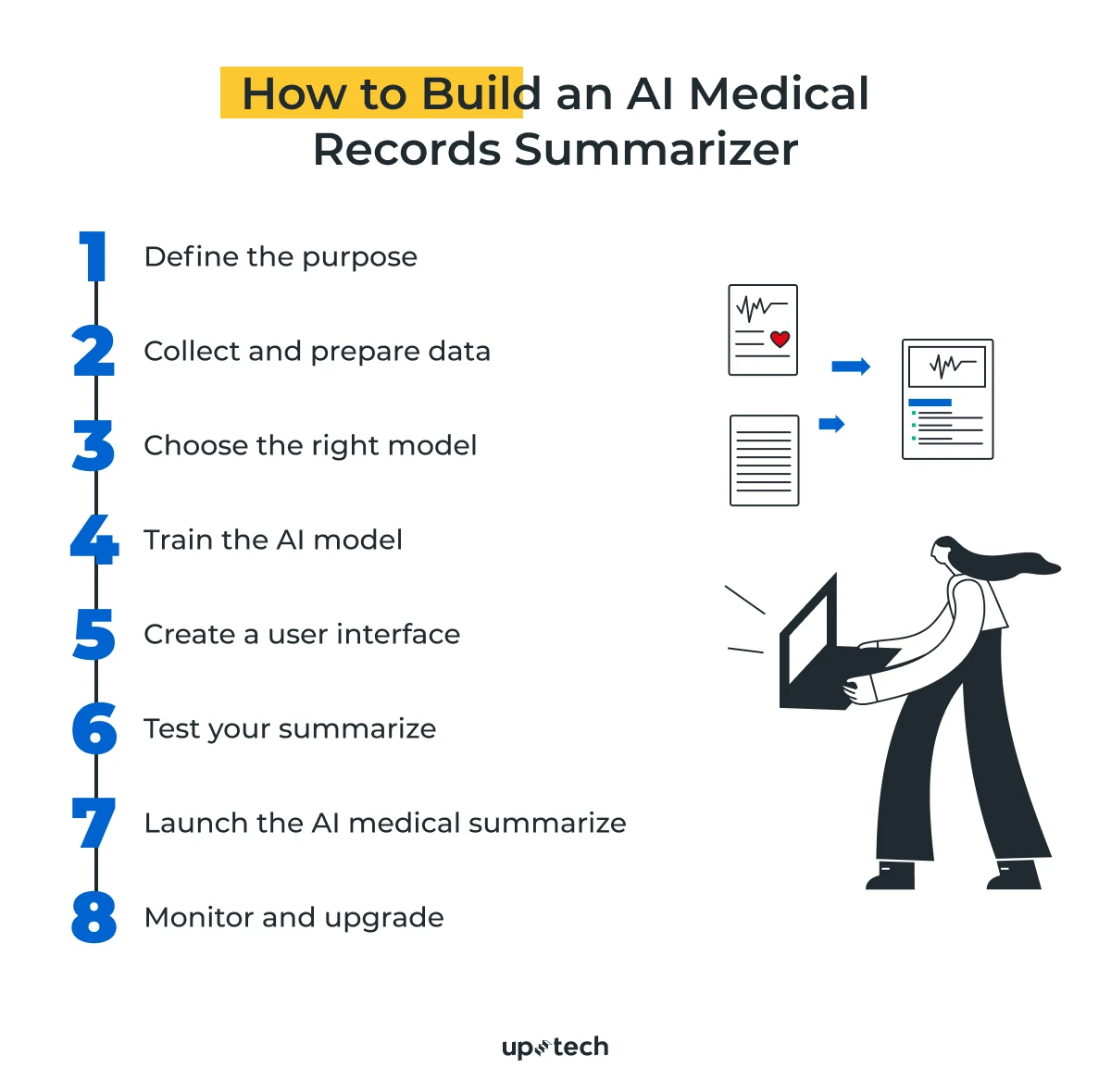
1. Define the purpose
Determine your product’s role in the medical record summary service it enables. Is it designed for extracting key information from medical imaging results? Or does it serve as an intermediary platform between insurers and patients?
Clarifying the app’s purpose helps your software teams decide on the right tech stack, business logic, and UI/UX elements.
2. Collect and prepare data
Data preparation is vital to producing accurate and high-performing AI apps. To do so, you’ll need to engage healthcare experts and a data annotation team. They will curate diverse training datasets that fairly represent the information the model will process in real-life.
3. Choose the right model
The best AI model for summarization isn’t necessarily a powerful large language model. Instead, choose a model that fits your app’s purpose. For example, if your app summarizes MRI images, you’ll need imaging models like VGG-16 or ResNet50.
4. Train the AI model
Existing AI models lack accuracy and correctness when inferring data it was never trained on. Therefore, you must train the model with your prepared medical datasets. We suggest fine-tuning pre-trained models already equipped with general NLP or image-analyzing capabilities. This way, you can save substantial costs and get your product on the market faster.
5. Create a user interface
Regardless of how powerful the underlying AI model is, users who feel disengaged when using the app seldom give it a second chance. So, designing a user interface that feels intuitive and works practically with good UI/UX elements is essential.
6. Test your summarizer
Run the summarizer through several test cases and look for signs of underfitting, hallucination, bias, and other performance issues. Fine-tune the model if necessary until it performs expectedly. Also, ensure the app integrates well with existing medical systems to prevent disruptions.
7. Launch the AI medical summarizer
Deploy the AI app in the production environment and watch for bugs or technical issues. Train and educate users about using the app for seamless onboarding.
8. Monitor and upgrade
Continuously evaluate the summarizer’s performance and ensure it aligns with healthcare practices. Implement upgrades when necessary to provide a secure, robust, and compliant AI app that solves real problems.
Practical Tips on Using Generative AI
Generative AI is still an evolving technology. The black box-like neural networks that deep learning models use make implementing GenAI challenging. Use these tips to reduce risks and challenges affecting the final product.
- Don’t compromise on the training sample’s quality because it affects the model’s performance. When training or fine-tuning a model, use a dataset that resembles what the AI summarizer will process in real life.
- Apply protectionary measures to the data you use for training the AI model. Similarly, ensure the entire data pipeline and infrastructure are secure and HIPAA compliant.
- If you use the summarizer for financial or arithmetic processing, be mindful that language models might produce erroneous calculations.
- Bias inherited from training samples can negatively affect patient outcomes. Ensure that the model is trained from a diverse and equal representation of the patients to reduce bias.
- Improve the model after deployment by training it with anonymized data generated from actual usages.
How can Uptech help?
Uptech is an international app development company servicing startups and enterprises worldwide. Our multi-disciplinary team is at the forefront of emerging AI technologies and has integrated genAI into several apps. A notable example is Hamlet, an AI app summarizing PDF documents we built for a US-based company.
.webp)
We’re also equally knowledgeable in the medical industry. Our experience in developing healthcare apps is helpful in architecting secure apps that comply with HIPAA and other regulatory acts. The intersecting expertise in AI and healthcare spaces put us in an excellent position to build an AI-powered medical records summarizer for you.
Through a proven development framework, we balance cost and development time for clients with sensitive budgets and market windows. For example, we can build a PoC for $20K in approximately 2 months. More importantly, we conduct a thorough discovery phase and survey your users to ensure a tight product-market fit.
But don’t take our words for it. Check out what our clients say on Clutch
“Uptech is a great partner for software and web development project”, - Executive, Angler AI.
“They ask questions, provide suggestions, and learn about our business in order to make a proper product.” - Co-Founder, Eatable.
Summary
Generative AI brings tremendous potential in transforming how healthcare extracts and summarizes information from medical records. It improves patient care delivery by improving efficiency and consolidating fragmented information in medical facilities. Despite that, there exist functional and regulatory challenges that solution providers must tackle when developing AI summarizers.
Choosing the right AI model, curating quality datasets, and continuous model assessment are crucial to delivering a functional AI app. I’ve shared ways that will help you build an AI medical summarizer. However, practical skillsets, regulatory knowledge, and experience in AI development are indispensable in bringing your ideas to life.
Discover more about building an AI summarizer with Uptech today.