Interface.ai recently secured $30 million in funding to supercharge how banks manage customer requests using artificial intelligence. Around the same time, One Zero, an AI-driven fintech founded by Mobileye’s creator, reportedly raised $100 million. These AI trends in banking only mean one thing: AI is becoming the backbone of banking innovation.
If you’re a fintech business owner, a banking executive, or just entering the financial industry, staying on track with AI and ML trends in baking is critical. AI and ML offer a range of opportunities — from unlocking data-driven insights and enhancing fraud detection to boosting brand engagement, improving customer experiences, and strengthening risk management.
This isn’t just theory. Industry leaders like JPMorgan Chase and Capital One have shown how AI can reshape financial institutions. Reports indicate that nearly half of all companies have widely embraced AI, and 43% consider it critical to their success by 2025.
In this article, Oleh Komenchuk, an AI&ML Engineer at Uptech, and Dima Kovalenko, CEO and co-founder of Uptech, will guide you through the latest AI and machine learning trends in banking and finance. With over 8 years of experience supporting fintech businesses and developing AI-based solutions, we’ll help you stay ahead in this competitive market.
Artificial Intelligence in Banking: What Is Happening in the Market?
The global AI market in banking was valued at $19.9 billion in 2023 and grew to $26.2 billion by 2024. By 2033, it’s expected to become as high as $315.50 billion. That’s equal to a strong annual growth rate of 31.83% from 2024 to 2033. This trend will only continue as more and more banks and financial institutions go digital and modern.

Here’s how far AI has already impacted the finance industry:
- Automation of transactions: In the UK, several banks now fully automate loan approvals for amounts up to $100,000.
- Fraud detection: NatWest has reduced fraud by 6% across the UK and achieved a 90% reduction in new account fraud since 2019. This improvement has cut costs and spurred higher interest in loans, with AI personalization resulting in 5x more clicks on offers.
- Productivity boost: AI is expected to raise productivity in investment banks by 27% and boost front-office productivity by 27%–35% by 2026. A recent McKinsey report also highlighted that generative AI could contribute between $200 billion and $340 billion annually to the global banking sector, primarily through productivity gains.
Looking ahead, predictions indicate that AI will drive further advancements in several areas. This could include deeper integration in decision-making processes, such as loan origination, credit assessments, or client relationship management. We’ll discuss these trends in the next section.
15 AI & ML Trends in Banking to Look Out for in 2025
As you’ve seen, both industry giants and agile startups are integrating AI and ML into their fintech software ecosystems. If you haven’t already, now is the time to strategize how to bring them into your business.
But where do you start?
Let’s explore 15 AI & ML trends in fintech to watch for in 2025.
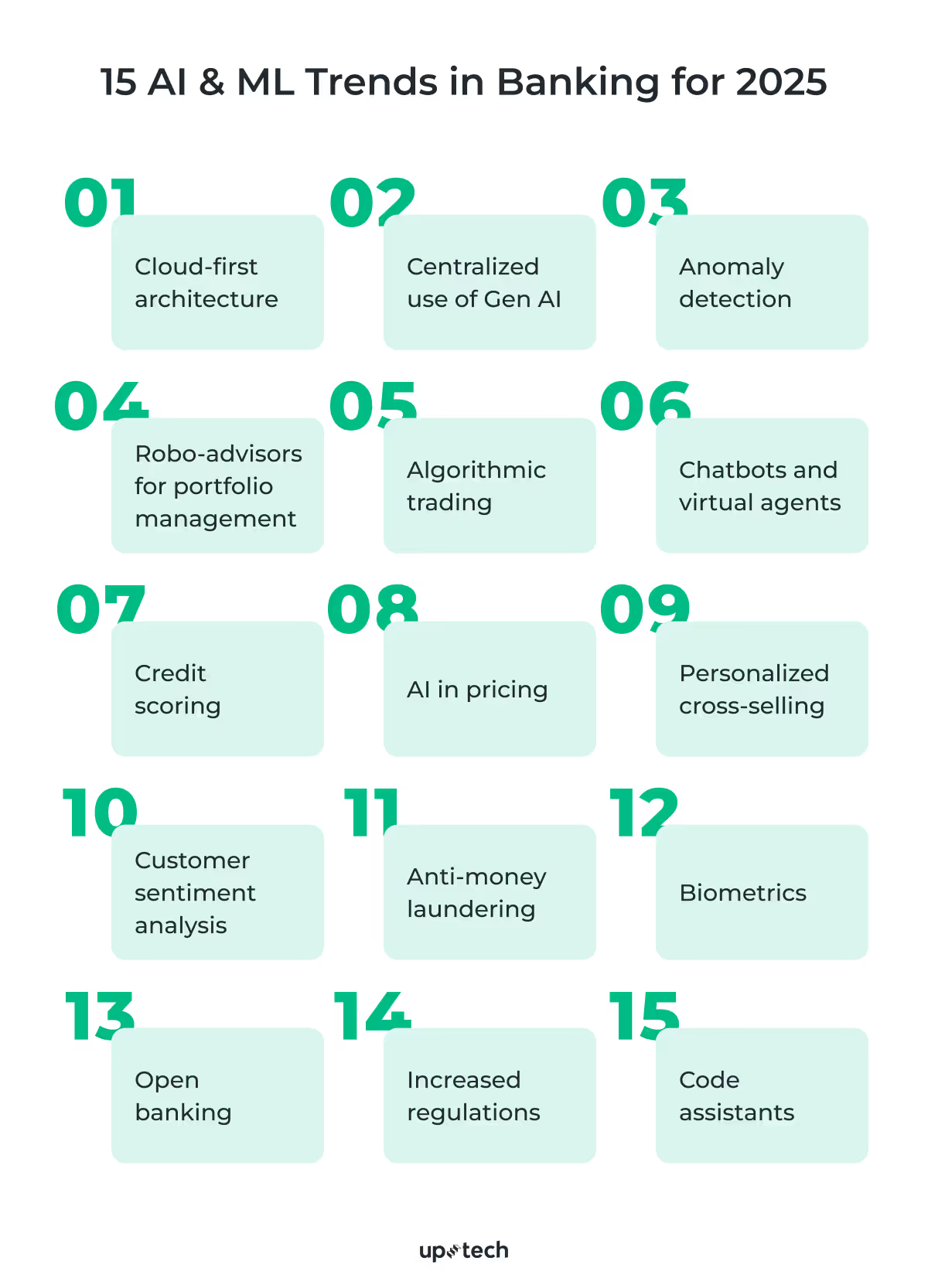
Cloud-First Architecture
Scaling is one of the many fintech challenges banks face, and the best way to tackle it is to adopt a cloud-first architecture. This approach is also important if you want to integrate AI into your banking application.
AI workloads require substantial computing power and data resources that are challenging, if not unmanageable, with on-premises solutions. That’s why we’ll see cloud computing becoming a critical strategy moving forward.
You can also consider a hybrid approach by prioritizing cloud-first while maintaining smooth connections between your cloud and on-premises systems to simplify migration.
If you’re wondering how to develop a mobile banking app, check out our comprehensive guide.
We’ve built solutions using popular cloud services like AWS, Azure, and GCP to create scalable apps for fintech and other industries. Tell us about your project, and our team will be glad to help you move forward.
Centralized Use of Gen AI
Generative AI in banking can be expensive, so financial companies need a way to scale their AI programs to fit their business needs. McKinsey’s study revealed that over 50% of financial companies, with assets worth around $26 trillion, centralize their use of generative AI systems to support wider deployment in their organizations.
When you adopt this setup, you’ll have a core team in charge of your company’s entire AI solution, which helps tackle challenges like:
- Incorrect or illogical outputs
- Intellectual property violation risks
- Complex system workings
- Bias or unfair outcomes
- Security risk management
Your core team will be able to implement rigorous quality control, standardized practices, consistent monitoring for bias, and comprehensive security measures. The AI system also remains uniform since only one team handles it, which avoids complexity when you adopt the system across your entire organization.
Anomaly Detection
In 2022, fintech startup Revolut’s database was breached by cyber attackers, which they unfortunately discovered late. Financial companies often struggle to identify unusual activities, including fraud, abnormal cash payments, account takeovers, network hacks, and money laundering.
If you have a business to protect, machine learning and robotic process automation can become vital tools in your cybersecurity arsenal. For example, you can use them to:
- Spot irregular patterns in your network activity indicative of unauthorized access or cyber-attacks
- Identify and flag behaviors or digital signatures linked to known cyber threats
- Enable real-time notifications for suspicious activity and automate responses like blocking malicious IPs or isolating affected systems
Robo-Advisors for Portfolio Management
Fintech companies compete on who will attract more customers with new and valuable functionality. If you’re in the investing space, you have the chance to offer robo-advisors — tools that provide automated financial advice using smart algorithms to create and manage investment portfolios.
66% of people in the UK feel nervous about investing, and apps with portfolio management features perfectly address that. A great example of this is Betterment, which uses robo-advisors to offer personalized portfolio management and automated investing. Similarly, Vanguard has integrated AI into its platform to optimize portfolio recommendations and reduce fees.
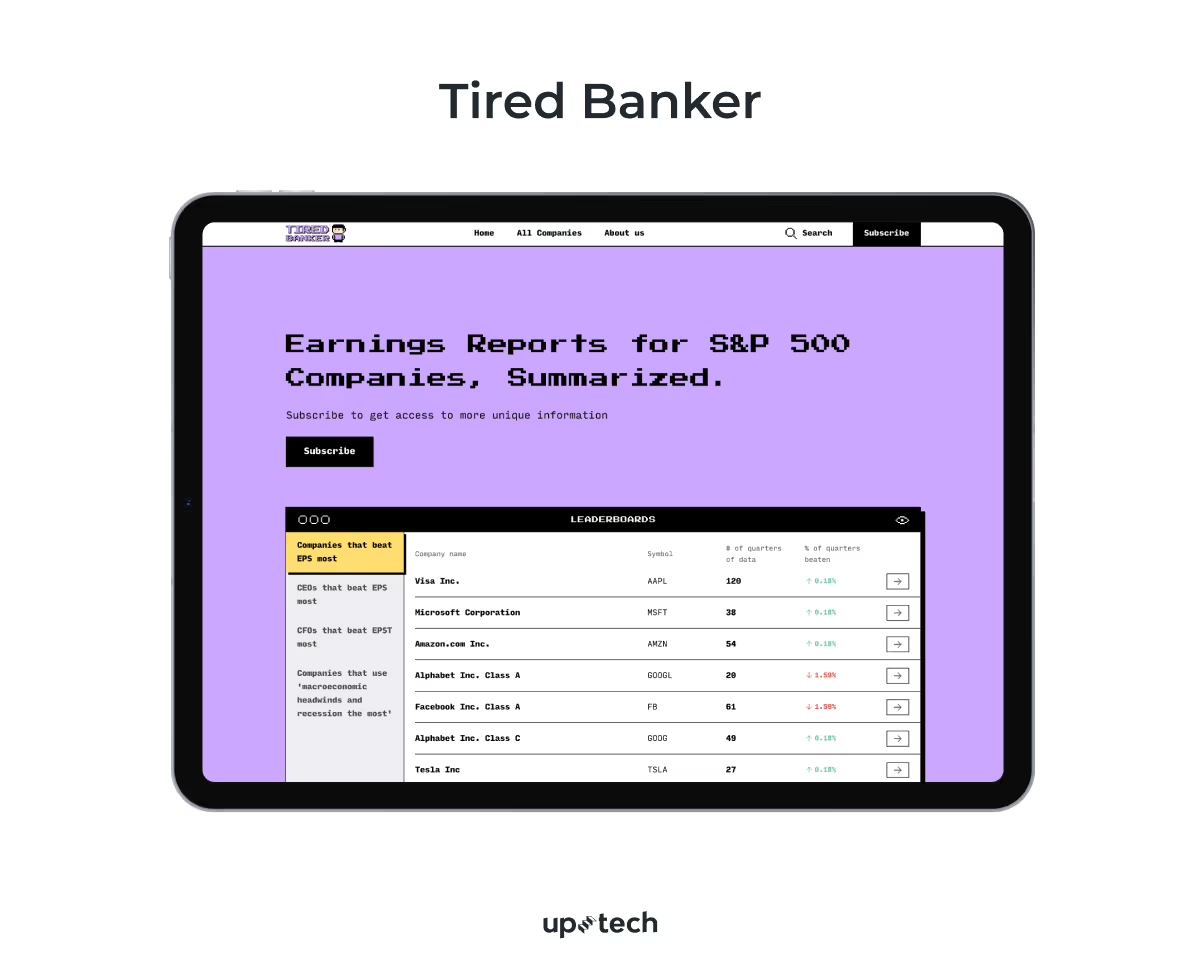
At Uptech, we worked on a similar platform called Tired Banker, which helps users understand complex investment data. The platform looks at earnings reports from the past 10 years of every S&P 500 company and turns the information into something simple to consume using GPT-4 technology.
Algorithmic Trading
Algorithmic trading simplifies the process of buying and selling securities by using algorithms to break large stock orders into smaller, manageable “child orders,” which are executed based on predefined rules like price, volume, or timing constraints.
Over the last 20 years, financial customers now account for around 50% of global FX trading, thanks to new trading platforms. Specifically, algorithmic trading now handles nearly 75% of spot FX transactions.
BNP Paribas is one great example of a company offering algorithmic trading services, customized to their client’s preferences, as part of their services. Among many benefits, they leverage AI to:
- Combine multiple data sources for a clearer view of the market.
- Automate trading decisions using predefined rules and ML models.
- Adapt and improve strategies as market conditions change.
- Real-time transaction monitoring and reporting.
If you have a trading app, adding algorithmic trading features could be a game changer for you and your users. Algorithms like decision trees, neural networks, and support vector machines analyze large datasets and learn from past market behaviors. They identify patterns you or your users might miss, which could enhance your trading decisions.
Chatbots and Virtual Agents
PwC found that 73% of consumers consider customer experience (CX) the most important factor when deciding whether to purchase from a company. One effective way you can improve CX is through the use of AI-powered chatbots and virtual agents.
AI chatbots can allow users to check balances, make transactions, and transfer money simply by chatting. Meanwhile, virtual agents can assist users in setting up new accounts. When more complex issues arise, these tools can direct customer complaints to the right departments. And the best part? They're available 24/7.
You can start simple in your chatbot and virtual agent integration, e.g., integrate ChatGPT in the finance app to enable instant responses to customer inquiries and tasks. A proven example is Bank of America’s Erica, which assists customers with everything from accessing account information to making payments and even receiving personalized financing suggestions.
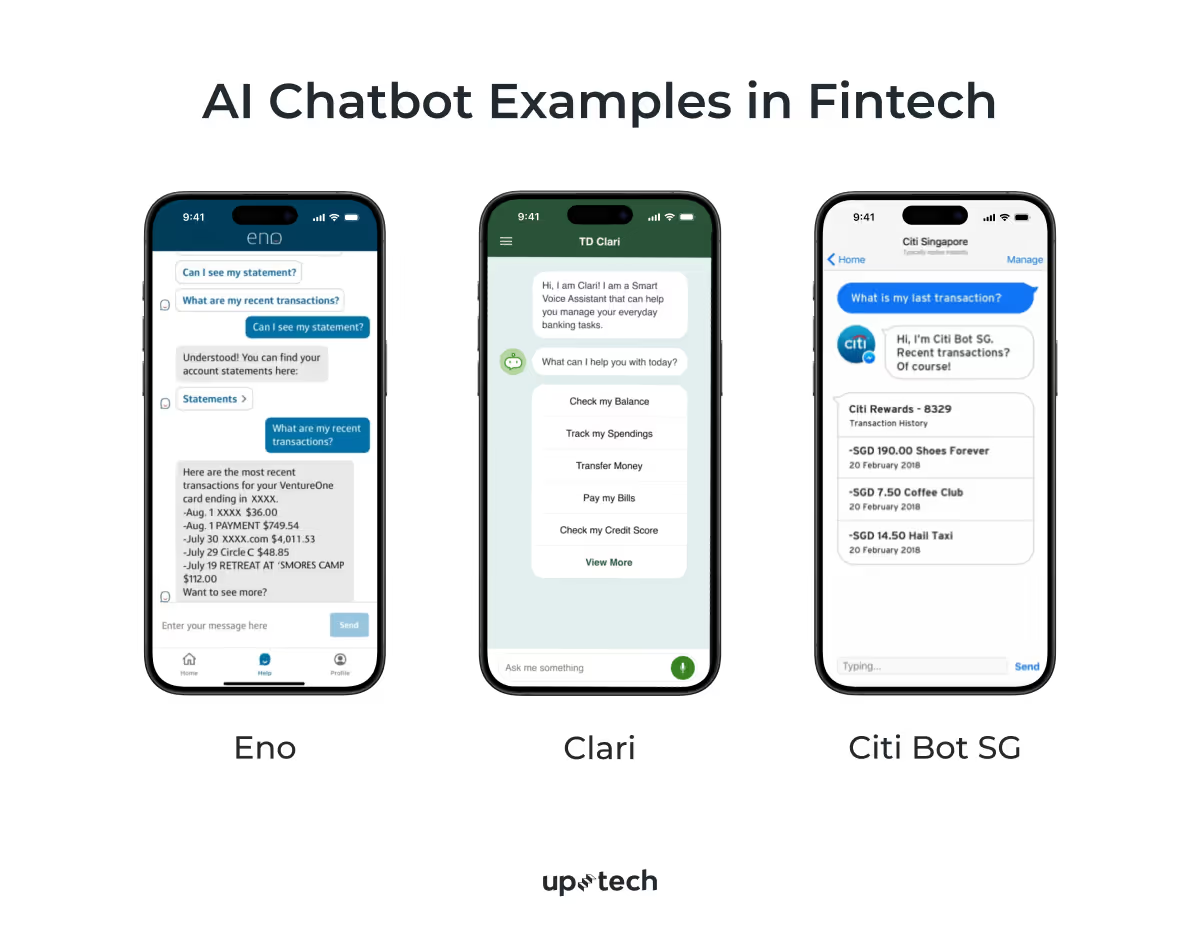
Capital One offers its own chatbot assistant, Eno, while TD Canada Trust has Clari to help users manage their finances. Citi also did great by launching the Citi Bot SG on Facebook Messenger back in 2018, giving customers easy access to support through social media platforms.
If you want to learn how to build an AI agent for your business, take a look at our knowledge resources or reach out for detailed help.
Credit Scoring
Credit scoring is definitely one of the biggest machine learning trends in insurance and finance. Since the data is handled using algorithms, you can make the credit scoring process fairer and more objective compared to human scoring methods.
Key advantages include:
- Applying for loans is quicker and easier — users can do it from home.
- The system speeds up the process of sorting, tagging, and processing documents.
- It eliminates biases that can affect traditional credit scoring.
- It can manage large datasets and handle many variables and their interactions, which is common in credit scoring.
- They can be easily updated as new data comes in, helping lenders adjust to market changes and evolving consumer behavior.
You can also combine machine learning with tools like optical character recognition (OCR) to process scanned documents more easily. This helps organize information for future use, which makes it faster to onboard new customers and improve their banking experience.
AI in Pricing
You can mix human expertise, generative AI, and a wealth of data to improve your scenario planning and create personalized pricing strategies. Typically, your relationship managers need to do the following:
- Gather key details to fully understand the client’s needs.
- Identify the most suitable products for the client while considering the associated risks.
- Use pricing tools to evaluate the expected returns and adjust them for risk.
- Discuss and agree on the deal price with the client.
With artificial intelligence, though, you can make these steps much easier. AI can act as a decision support system for your managers. When equipped with the right data, AI provides real-time insights and recommendations for pricing. This makes it easier to structure prices effectively while still ensuring you’re making a profit.
Personalized Cross-Selling
One of the main AI trends in insurance and banking is offering customers products with AI personalization. AI tools can look at things like transaction history and spending patterns to suggest the best financial products, like credit or debit cards, for each person.
With AI and machine learning, banks can:
- Study customer behavior
- Predict what customers might need next
- Adjust offers in real-time based on customer preferences
For instance, an AI assistant can notify customers about the latest card offers and explain how these cards align with their financial goals. Hyper-personalization and AI make the experience more enjoyable for customers and increase their chances to choose additional offers from your company.
Wells Fargo has already done it with their Fargo assistant, which helps customers track spending, predict expenses, and make smart financial moves. Similarly, Morgan Stanley uses Debrief, a tool that generates meeting notes and action items for advisors, which can be helpful in personalizing client offers.
Customer Sentiment Analysis
Customer sentiment analysis is one of the most important banking trends as it involves personalization. Sentiment analysis is a method used by AI to analyze text and determine whether it expresses positive, negative, or neutral feelings. It’s especially useful if you want to understand how customers feel about your financial services and products.
For example, DataEQ’s UK Challenger Bank Sentiment Index analyzed over 118,000 tweets about banks like Monzo and Atom Bank. It showed Monzo and Atom Bank had more positive feedback, thanks to their responsive customer service, while other banks struggled with complaints about slow turnaround times.
This analysis helps banks improve services and tailor offerings based on customer sentiment. You could apply the same analysis to yours.
AI also helps you by:
- Analyzing unstructured text data to derive actionable insights.
- Assigning scores to customer feedback to classify it as positive, negative, or neutral.
- Identifying the main topics in customer feedback.
- Recognizing specific products, services, or companies mentioned in feedback.
Anti-Money Laundering
AI and ML transform banking security with enhanced detection of suspicious activities — money laundering in particular. More banks are starting to use these tools to spot unusual transactions with speed and precision.
One notable example is Google Cloud’s Anti-Money Laundering AI (AML AI), which helps banks improve risk assessment by generating monthly risk scores for retail and commercial customers.
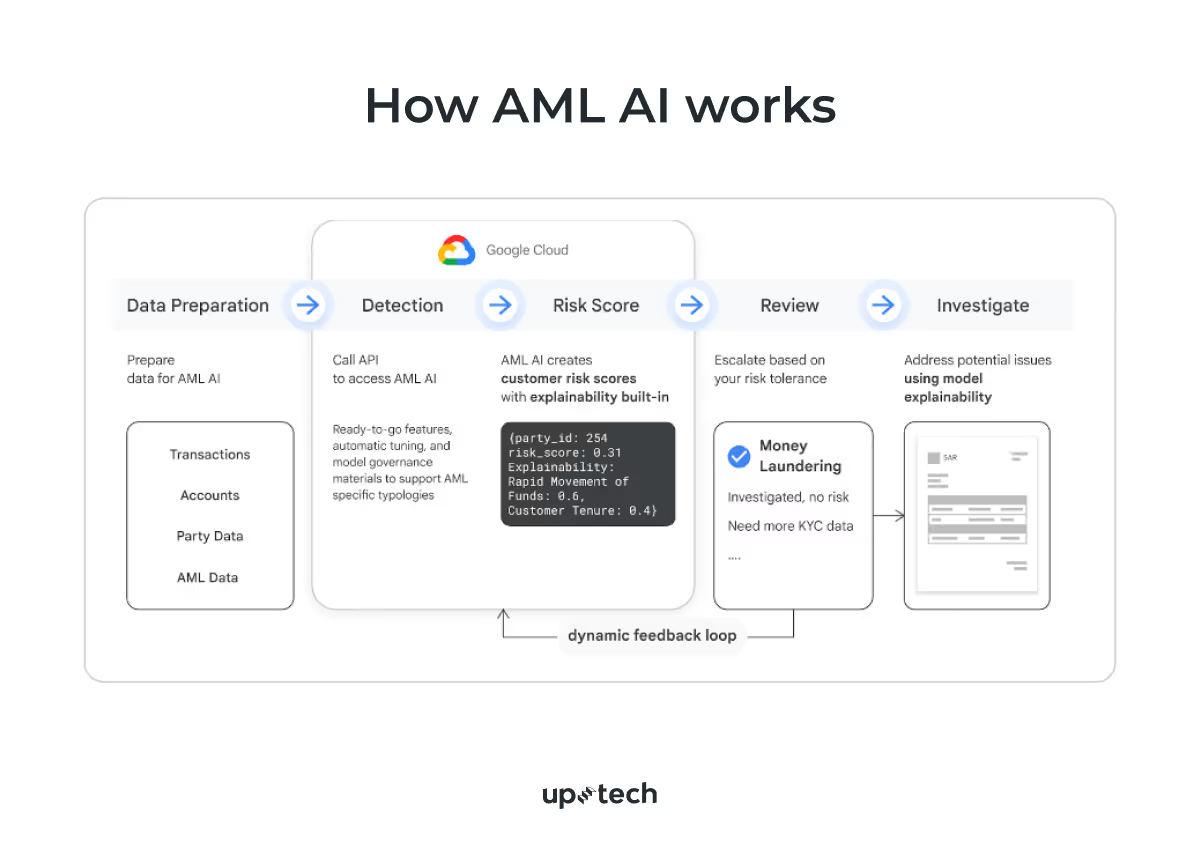
It offers clear insights for analysts, risk managers, and regulators and can integrate with or replace older systems. Additionally, AML AI allows banks to add custom risk indicators and complies with strict governance standards for risk model management.
As measured by HSBC, Google Cloud’s AML AI detected 2 to 4 times more confirmed suspicious activities and cut false positives by over 60%. This allows banks to spend more time investigating serious risks while improving security for everyone.
Biometrics
Biometric data, such as fingerprints, has been a key part of multi-factor authentication for years. Thanks to AI advancements, biometrics now extend to voice and facial recognition, which delivers smarter and more secure authentication methods.
Capital One’s voice recognition system is an excellent example of this.

When a customer makes an in-store payment using their mobile phone, the system checks if the phone’s location matches the store’s. It will now provide a CAPTCHA and prompt the customer to read it aloud. The system records the voice and compares it with a stored sample. If the voice matches, the payment is approved.
Looking ahead, AI-driven biometrics promise even faster, safer, and more seamless banking experiences.
Open Banking
Open banking is revolutionizing the financial industry by enabling banks, fintechs, and third-party providers to securely share data through APIs. A study by Accenture shows that 76% of banks anticipate open banking usage to grow by more than 50% by 2026.
So, where does fit into this?
- Real-time monitoring of transactions is crucial in open banking. You can use AI to check unusual activity, spot real threats, and reduce false alarms.
- With customer data from open banking, AI can recommend financial products that match each customer’s needs.
- AI analyzes vast amounts of data from open banking to find patterns in customer behavior, market trends, and risks. Banks can use these insights to make better decisions and work more efficiently.
At Uptech, we specialize in integrating API for fintech apps. Our team has successfully partnered with a US company on open API integration, so we know how to handle the process step by step.
Increased Regulations
AI is becoming more important in the financial world, not just for banks, but also for legal and regulatory bodies. We understand that this news might be a bit unsettling, especially if you’re new to fintech. But the role of these institutions is to make sure AI is used safely, so it doesn’t put anyone’s personal or financial data at risk.
In Europe, the EU AI Act was published in July 2024. Some parts of this law, including provisions on prohibited AI practices, are expected to be applied as early as February 2025.
Meanwhile, in the US, the AI Bill of Rights was introduced at the end of 2022. It outlines five important rights that should be followed when using AI in all industries, including finance and banking.
Your main priority now is to ensure your AI integration is secure and compliant with all regulations.
To achieve 100% compliance, you can partner with a top AI development company like Uptech. When we work on AI products, we aim to apply all the regulations and be transparent with users about the data the product needs to reach its potential.
Code Assistants
According to Gartner, by 2028, 75% of software engineers will be using AI code assistants, up from less than 10% in 2023, thanks to generative AI. This shift is also set to modernize outdated banking applications.
AI code assistants can translate legacy code into newer programming languages with simple instructions. They can also identify and resolve errors efficiently. Further, you can actually leverage them to generate and run tests for better reliability. As such, it will be much much easier for you to upgrade older applications, prioritize updates, and rewrite code where needed.
Back in 2023, Gartner also predicted that 60% of the data used for AI would be synthetic by this year, meaning it is created instead of being collected from real-world sources. This synthetic data helps train AI models more effectively, minimizes errors, and reduces the risks associated with handling real-world data.
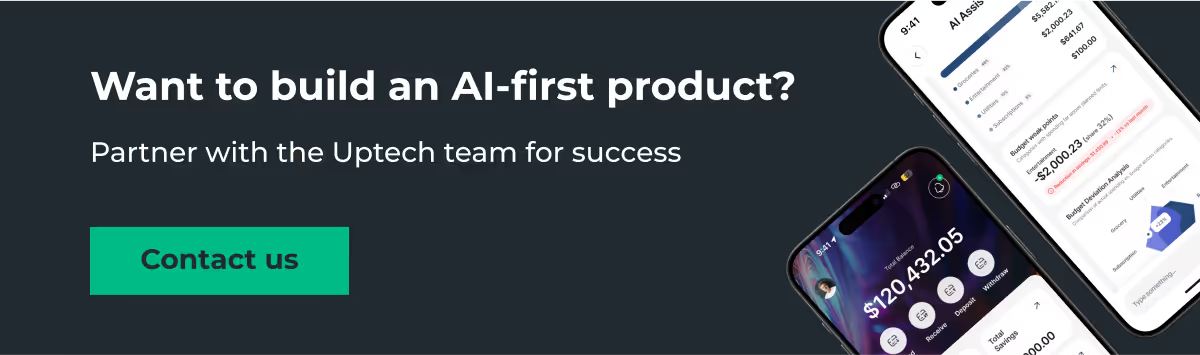
How Should Business Successfully Follow AI & ML Trends in Banking?
Before getting carried away by these trends, take a step back and think about whether it’s the right move for your business. AI has huge potential, but you need to consider how it aligns with what you really need.
Below, we’ll walk through ways to bring AI into your business in a way that works for you and sets you up for smooth, secure implementation.

1. Decide on the AI Trend to Implement
You don’t need to implement all AI trends at once — doing so could be a waste of resources if it doesn’t work for your business. Start small by focusing on one area where AI can make the biggest difference, like improving efficiency or enhancing the customer experience. For instance, automating customer support with a chatbot could be a good first step.
The best way to decide on that is through product discovery, and we can help you with that. We’ll first validate your idea, analyze your market, and find the product-market fit to ensure that we build the right product to address your business needs.
2. Consult with AI Experts
Aside from learning how to integrate AI into an app, you should also consider things like cloud storage, data management, and how everything will work together. As such, engaging with AI experts familiar with the banking sector can streamline the process.
At Uptech, we’ve worked on various banking platforms, including investment portfolios and card management systems. Our expertise helps clients choose the right technologies, AI tools, and deployment strategies. We also create customized AI roadmaps aligned with business objectives and regulatory requirements.
3. Outsource AI&ML Expertise
When you’re ready to implement your AI project, it’s time to find a reliable AI/ML outsourcing provider. Outsourcing is often more cost-effective than building an in-house team, and you get practically the same results. This way, you can:
- Scale your AI capabilities while keeping quality and security intact
- Get faster results and high-quality, expert-driven solutions
- Free up your internal team to focus on other critical business tasks
At Uptech, we offer fintech software development services, including model customization, deployment, and ongoing support. Our experienced AI team creates tailored solutions to meet your specific business objectives. We offer a brunch of services related to AI, from consulting to computer vision development:
- Generative AI consulting services
- ML development solutions
- AI chatbot development services
- Computer vision services
4. Ensure Security and Compliance
It’s also important to focus on data security and follow the rules. Since financial data is highly sensitive, banks must adhere to industry regulations such as GDPR, the EU AI Act, and local financial laws. Work with your security and compliance teams to build strong AI fintech systems that are clear, ethical, and can spot and reduce risks like fraud and bias.
A simple way to start is by building a strong data system. You should clean, organize, and collect high-quality data from different sources. Doing this helps create better predictive models, too.
Here’s our guide to fintech security and a checklist you can download.
5. Implement Responsible AI Practices
Once your AI system is up, make sure your business consistently follows responsible AI practices. Remove any biases from your algorithms, especially when dealing with diverse populations. It’s also crucial that AI decisions are explainable — this helps customers and regulators trust the outcomes. Inform your clients about data collection policies so they know how their information is being used.
Consistent monitoring is key. Regularly assess AI performance to ensure it remains ethical, compliant, and aligned with your business goals. Also, make sure your team is updated with evolving regulations like GDPR or emerging industry standards to avoid risks like bias or fraud. We know from our experience that keeping AI practices responsible will build customer trust and ensure you meet regulatory expectations.
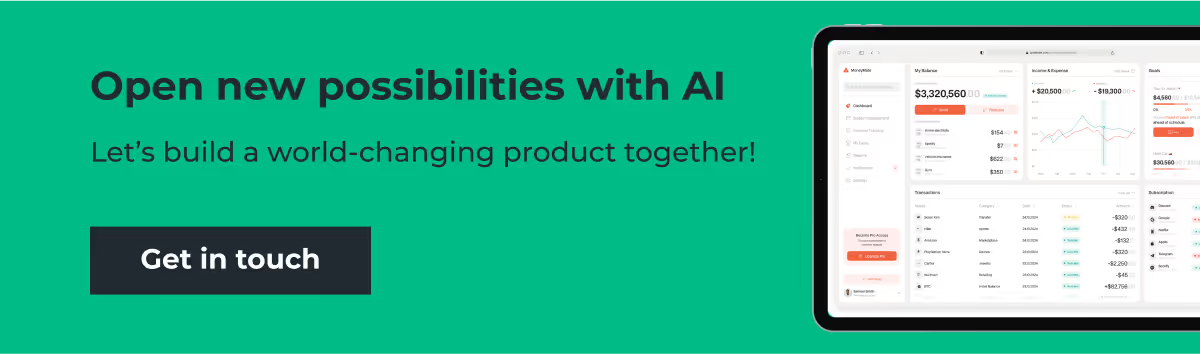
Trending AI Technologies to Apply for Banking
As a machine learning engineer, I’m thrilled by how AI is reshaping industries, especially in projects we’ve worked on at Uptech. For instance, Hamlet uses AI to summarize tons of text. There's also AnglerAI – an AI-powered platform for customer acquisition and customer lifetime value, and Dyvo.ai for image and avatar generation.
But this is just scratching the surface. AI’s potential for the banking industry is immense. Let’s take a look at some of the latest technologies defining AI in banking today.

Retrieval-Augmented Generation (RAG)
RAG is gaining traction in banking, particularly for tools requiring highly accurate information. It connects generative AI to a knowledge base, which allows systems to access and process vast amounts of financial data.
As a result, you get more precise and context-aware responses.
This technology makes generative AI more reliable, particularly for banking tasks that require detailed background knowledge, such as financial planning or compliance checks.
Multimodal Models
Multimodal models can handle different types of data at the same time, such as text, images, and audio. When used with AI-powered tools like virtual agents, they create more interactive and user-friendly banking services.
These models are beneficial for tasks that combine various data types, like reviewing financial reports, analyzing customer feedback, or processing and verifying transaction records.
Lightweight, Optimized Models
You don’t want your AI to become impractical. Lightweight models help ensure advanced AI stays useful, even in environments with limited resources. These models can work smoothly on mobile devices and edge systems, making them more accessible.
Developers often use techniques like quantization and model pruning to minimize resource demands while keeping performance and accuracy intact. For banking apps, this means more accessible AI-powered tools, such as chatbots, mobile investment platforms, efficient document processing, and personalized financial services.
Fine-Tuning With Personalization
You can use open-source tools to personalize AI models for your specific needs, like detecting fraud or offering customer support.
Instead of creating a new model from the ground up, you can improve existing pre-trained AI models. This saves time and money while providing you with the right tools to enhance customer service, manage risks, and streamline financial tasks.
As a result, customers enjoy more personalized services, and your operations become more efficient.
Integration With No-Code/Low-Code Platforms
AI is being added to no-code and low-code platforms, which makes it easier for businesses to leverage the power of AI. These platforms allow banks to create apps or automate tasks with minimal coding.
With AI built in, they can use tools like chatbots or personalized customer service systems, even without developers. While this is great for startups, we recommend consulting with experts and seeking professional development services, especially when dealing with sensitive financial data. Drop us a line and we’ll be happy to learn more about your project and give a free consultation.
FAQs
What is ML in finance?
Machine learning in finance is about creating smarter, more responsive systems that can process and learn from vast amounts of data — like transaction records, market activity, and customer interactions. By recognizing patterns and making predictions, it helps financial companies stay ahead, whether it’s spotting fraud, forecasting trends, optimizing investments, or better understanding customer needs. It’s not just about efficiency; it’s about rethinking what’s possible when technology supports human decision-making.
What is AI in banking?
AI in banking is transforming the way financial institutions connect with people and solve problems. It uses intelligent systems to analyze data, detect fraud, and streamline operations — but its real power lies in creating more meaningful, personalized experiences. Whether it's helping customers make smarter financial decisions or simplifying complex processes, AI isn’t just a tool; it’s a way to reimagine how banks can better serve individuals in a fast-changing, digital-first world.
What are the benefits of AI in financial services?
The benefits of AI in financial services depend on the use case but generally include:
- Boosting operational efficiency
- Reducing manual errors
- Cutting operational costs
- Strengthening risk management.
- Offering tailored financial products based on customer needs
What are the use cases of AI in banking?
AI applications in banking enhance fraud detection, automate customer support with chatbots, and boost credit scoring accuracy. Banks also leverage AI to personalize financial advice, recommend tailored products, and speed up loan approval processes. It can also strengthen risk management strategies thanks to improved AI-driven security measures.
What are the challenges of AI in banking?
AI in banking holds incredible promise, but it comes with its share of challenges. Ensuring data privacy and security is critical in building trust, while navigating complex regulations adds layers of responsibility. Bias in AI models can lead to unintended outcomes, this puts the need for fairness and transparency on top. And as systems become more automated, there’s a fine balance to strike between efficiency and maintaining the human connection. The challenge isn’t just about adopting AI—it’s about doing it in a way that’s ethical, inclusive, and deeply attuned to the needs of people.