Computer vision has emerged as one of the driving forces in the technological space. While computer vision dates back to the 1960s, recent artificial and deep learning advancements propel the technology to greater heights. Notably, medical professionals are applying AI-powered computer vision in healthcare to improve patient outcomes, streamline treatment, and reduce costs.
As startup founders and product managers, you might wonder if a sustainable market for computer vision medical products exists. Fear not. According to statistics, the global computer vision in healthcare market is expected to grow from $0.71 billion in 2021 to $5.15 billion in 2026, at a 48.92% CAGR. The forecasted growth underscores the transformational effects that deep learning and generative AI technologies bring to the fold.
At present, information overload might cloud your decision to pursue interests, implementation, and investments in healthcare computer vision applications. As Uptech co-founder, I’m here to clear your doubts about exploring, developing, and expanding products for medical computer vision use cases.
Our team has stayed abreast with generative AI technologies, applying deep learning and computer vision models in our works. For example, we built Dyvo.ai, an app that turns selfies into AI-generated social avatars, which we later expanded into Dyvo for Business and Dyvo Headshots.
In this article, I’ll explain how computer vision works in healthcare, its benefits, and how we can help you build such solutions.
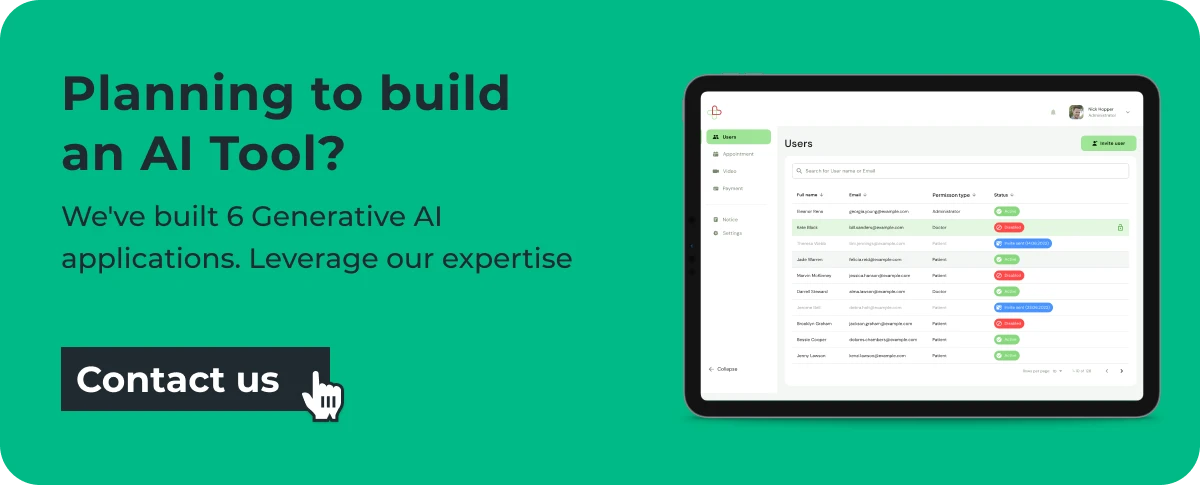
What is Computer Vision in Healthcare
Computer vision is a term that broadly describes the use of advanced technologies to replicate human capability in understanding and reconstructing visual images. In recent days, deep learning algorithms like convolutional neural networks are redefining how computer vision technologies work. For example, MidJourney, the popular generative AI service, can produce realistic images from text input.
The healthcare industry is no stranger to computer vision applications. CT scans, MRIs, and X-rays incorporate computer vision technologies to a certain extent. For years, doctors have used software to analyze imaging scans, diagnose patients, and prescribe subsequent treatments. However, generative AI greatly elevates computer vision’s accuracy, enabling use cases in areas previously impossible.
By training deep-learning AI models with large volumes of image data, computer vision systems can perform with up to 99% accuracy. This significantly improved from the 50% accuracy that legacy computer vision systems charted. The breakthrough provides strong motivations for discovering new use cases for vision-aided applications in the healthcare industry.
Noting the growing importance of computer vision in medical imaging, Nvidia has launched Clara-AGX, a scalable computing architecture that supports real-time image processing for medical use cases. Meanwhile, Medtronic provides an AI-enabled app that allows surgeons to prepare and practice procedures digitally.
Benefits of computer vision in medicine practices
Computer vision application has proved beneficial for medical professionals. Integrating generative AI will further strengthen the advantages of computer vision in healthcare.

Improve medical treatments and procedures
Computer vision in healthcare systems can improve procedure efficiency and reduce reliance on manual intervention. Instead of being fully hands-on when diagnosing and treating patients, clinicians use medical computer vision solutions to diagnose, treat, and support patient recovery. For example, radiologists can read CT scans faster with machine learning-enabled imaging solutions.
Accelerate healthcare research
Medical researchers spend hours studying images to analyze cancers, cardiovascular, and other diseases. Applying computer vision and machine learning algorithms speeds up the effort to understand pathological diseases and formulate treatments. Deep learning algorithms can ingest and process large volumes of data rapidly. More importantly, AI models can correlate patterns that might escape human eyes.
Improve overall patient experience
With medical computer vision, patients can afford a broader range of remote treatment options. Embedded computer vision models in patient monitoring systems allow doctors to continuously monitor recovery progress without being physically present. Patients will also benefit from automated reporting and in-depth visualization that better support their medical care journey.
Enables better healthcare decisions
Surgeons, clinicians, nurses, and medical staff face constant pressure to decide treatment options wisely to ensure optimal patient recovery. Yet, the influx of imaging diagnosis data and a narrow time window make such decisions challenging. Computer vision models can augment their assessment by providing unbiased analysis and inference based on imaging results.
Computer vision use cases in healthcare
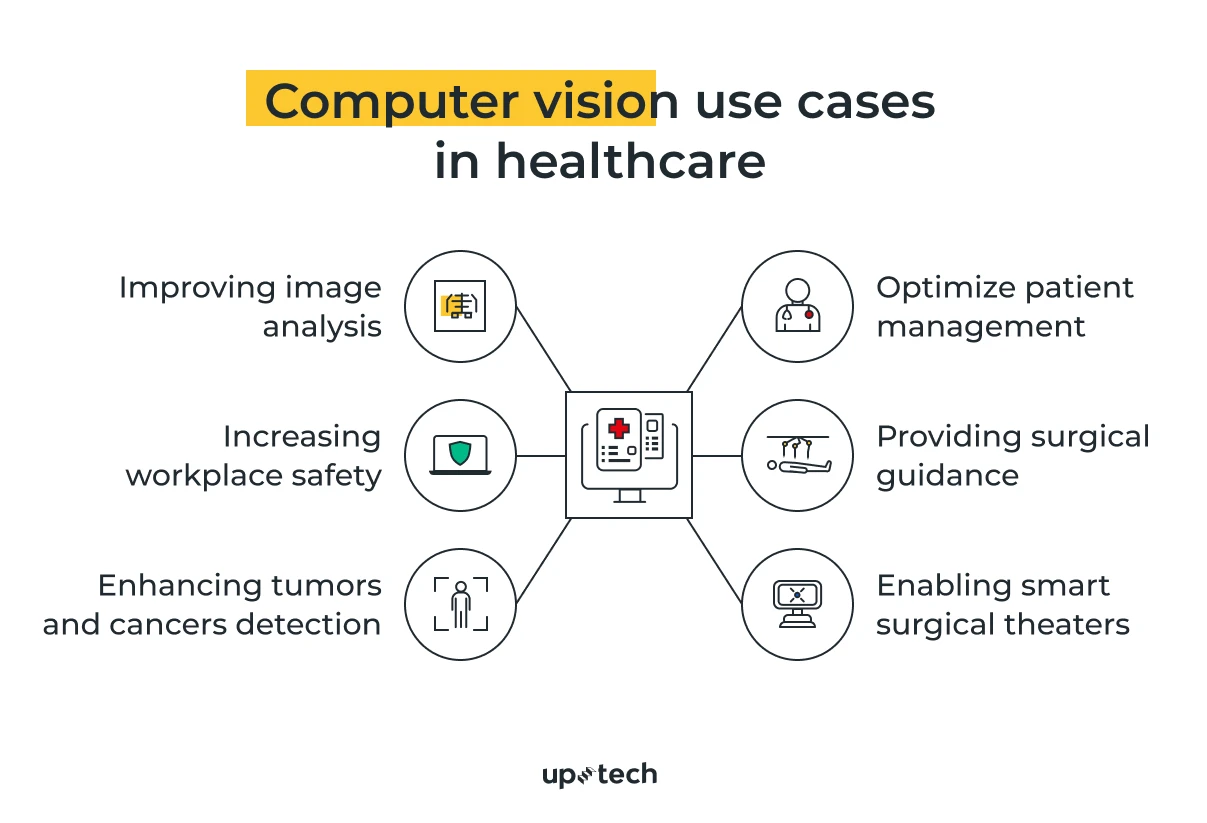
Deep learning computer vision technologies are more than short-lived hype. Rather, they are practical solutions that help healthcare providers improve medical care delivery, reduce costs, and manage risks more intelligently. Here’s how.
Improving image analysis
Computer vision systems, particularly those powered by deep learning algorithms, promise accurate prediction that helps radiologists in their tasks. Moreover, computer-aided scans produce faster results, allowing ample time for doctors to devise subsequent treatments.
Enhancing tumors and cancers detection
Some malignancies are incredibly difficult to detect until they progress to a later stage. With AI and machine learning, early detection can enable patients to receive treatment before a more severe prognosis. For example, medical researchers at the NCI’s Center for Cancer Research have developed a multiparametric AI model capable of accurately detecting prostate cancer early.
Optimize patient management
Prompt and precise treatment is essential to ensure the patient’s well-being. However, patient mix up might complicate healthcare provider’s attempts to deliver the best care they can. With computer vision, hospitals can integrate facial recognition with patient monitoring systems, ensuring accurate identification of patients and keeping track of their whereabouts.
Enabling smart surgical theaters
Surgeons performing procedures on their patients rely on visual guidance to minimize procedural risks. Computer vision in healthcare can provide real-time feeds on the affected area, eliminating mistakes such as accidentally leaving medical objects in the patient’s body. Besides, computer-aided systems allow medical staff to seamlessly document the procedure. Instead of manually feeding visual data to the EHR platform, surgeons and nurses can fully focus on the procedure.
Increasing workplace safety
Mishaps may happen at healthcare facilities, affecting patients and medical staff. Computer vision systems provide continuous monitoring, relaying alerts when detecting abnormal incidents. For example, medical staff might forget to wear protection suits when entering isolation rooms, or patients could fall into the wards. With AI visual detection and alerts, healthcare providers can take prompt remediation actions.
Providing surgical guidance
Surgeons could benefit from the visual clarity that computer vision systems provide. While still in its infancy, medical researchers are considering using visual-aided procedures, particularly for minimally invasive surgeries. For example, surgeons can analyze video from an endoscopic camera in real time to aid the procedures.
Adoption area in healthcare
As deep learning technologies continue to evolve, medical professionals contemplate the potential of AI-enabled computer vision in medicine.
Cardiology
Computer vision helps physicians manage patient risks with automated cardiological scans, including vascular imaging, CT prognosis, and MRI segmentation. Guided by AI, physicians can provide a more accurate and prompt diagnosis, allowing early intervention of detected anomalies.
Ophthalmology
Deep learning machine vision enables physicians to treat retinal issues accurately and improve outcomes. For example, patients will benefit from the advantages that computer vision offers to retinal scans, cataract surgery, glaucoma detection, and more.
Dermatology
When trained, a deep learning vision model can classify skin conditions accurately. This proves helpful for treating patients suffering from various skin diseases, sensitivity, or tissue injuries. In an interesting development, MIT researchers successfully detected early-stage melanoma with a deep convolutional neural network.
Orthopedics
Machine vision acts on visual data streams from imaging scans to analyze and reconstruct bone and muscular structures into 3D representations. It provides insights that help surgeons determine the best procedural practices and tools when remediating a patient’s conditions.
Radiology
Integrating computer vision with imaging devices allows radiologists to perform more scans and compile results in less time. Machine learning models can automatically detect, segment, and classify suspicious areas in X-rays, MRI, and CT scans. This way, the radiologist can escalate the results for further deliberation with appropriate specialists.
.webp)
Addressing HIPAA Compliance Concerns
When developing computer vision for healthcare use cases, companies face challenges complying with HIPAA requirements. HIPAA is a regulation that American health authorities impose to safeguard patient’s privacy. However, most deep learning models hosted by AI providers do not include HIPAA compliance.
OpenAI, which provides API access to its Chat API services, specifically implies that using its model for HIPAA-regulated applications is strictly prohibited. You can't market your solution to the US if you use third-party AI and computer services not designed for HIPAA use cases.
The immediate workaround is initiating a Business Associate Agreement with OpenAI if you plan to use their APIs. To do that, you must prove that you’ve previously developed similar healthcare products that comply with HIPAA. In this regard, Uptech has a stellar record in designing healthcare apps that meet stringent US regulatory requirements.
Alternatively, download open-source deep learning models like DINOv2 and host them on cloud infrastructure architected for healthcare services. Major cloud providers, such as AWS and Microsoft Azure, feature protectionary measures that safeguard data against adversarial risks. Coupled with security best practices that our team applies, you will have a medical computer vision product that meets HIPAA requirements.
How Uptech can help
Uptech is an AI app development company with more than 7 years of proven track records. Our team of software experts serves clients worldwide, delivering digital products that prove helpful for their audience. We’re both product and customer-centric, striving to bridge the market gaps our clients operate in.
.webp)
Several companies have partnered with Uptech to integrate AI with new or existing solutions. For example, we developed Hamlet, an app that uses a language model to extract and summarize text or PDF documents. We combined state-of-the-art AI technologies with proven software frameworks to deliver a scalable summarizer for our client.
.webp)
Equally important is our experience developing digital solutions for the healthcare industry. We architectured a mental health app for the US market, complying with HIPAA and other regulatory measures required. Besides robust data protection, the app provides features that users demand, including in-app messenger, scheduling calendars, and learning modules.
Uptech’s wealth of experience in both AI and healthcare puts us in an ideal position to bring computer vision in medical field and make your app stand out. We balance cost, time, and quality to help clients remain competitive while delivering exceptional products. In typical cases, we can build a PoC that costs approximately $20,000 in 2 months. This way, you’ll have clarity if the product has an actual demand before moving forward.
Summary
Computer vision technologies have been pivotal in advancing healthcare deliveries. With deep learning algorithms fueling recent developments, the future looks promising for medical practitioners and patients alike. From bettering patient outcomes to facilitating medical research, computer vision in healthcare applications prove helpful across the entire healthcare ecosystem.
Talk to our team now to build your healthcare computer vision product and comply with HIPAA requirements.