The insurance industry is gradually shifting away from the one-size-fits-all solution for policyholders. Cloud computing technologies introduce the possibility of personalizing insurance plans, enabling automation and other means that leverage data-driven insights. Adopting generative artificial intelligence (AI) in insurance will boost such efforts.
While the use of generative AI in the insurance industry is still in its infancy, solution providers are buoyed by the promises the technology holds. According to Statista, the global generative AI market is projected to hit $207 billion in 2030, charting a 24.40% compound annual growth rate (CAGR) in the coming years.
Integrating generative AI with your existing insurance systems or developing new ones seems to be the right move at this juncture. But it’s wise to pause and understand the challenges you must overcome to successfully implement AI products in a tightly-regulated industry..
As the tech lead and co-founder of Uptech, I’ve led our team to complete a series of AI-powered products. For example, we built Dyvo, an app that uses deep learning models to create vivid avatars from selfies. Encouraged by its success, we expand Dyvo’s use cases to ecommerce and studio photography.
In this article, I’ll share how generative AI will add transformative value when applied to insurance. More importantly, I’ll discuss challenges and strategies to develop functional AI solutions for insurance.
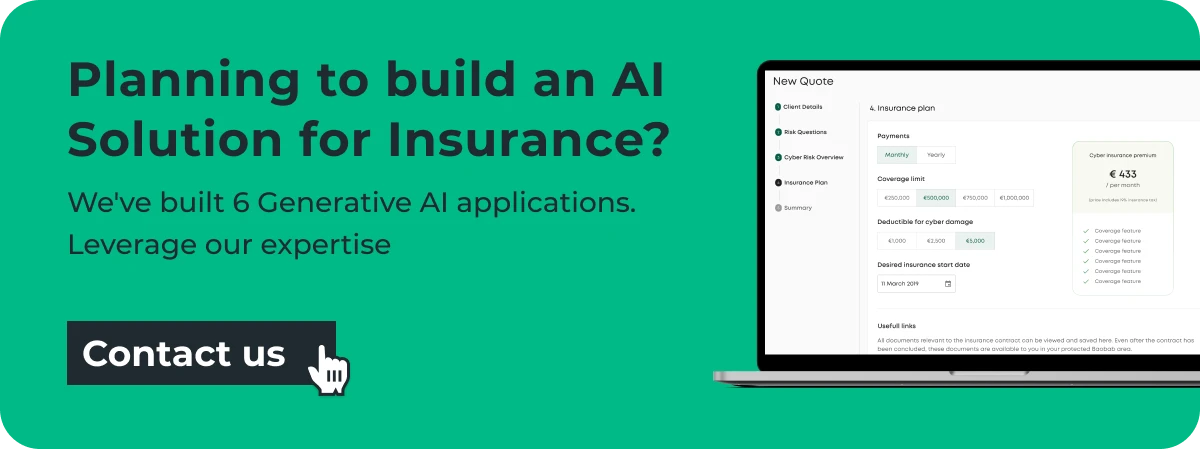
How Generative AI will Disrupt the Insurance industry
Generative AI is characterized by the technology’s ability to generate new, unique content with deep learning models. Deep learning is an approach in data science that trains neural networks with massive datasets. This allows the machine learning model to mimic human thought processes when predicting probable outcomes. For example, ChatGPT is a generative AI system capable of writing poetry in styles similar to human authors.
In the insurance market, generative AI can leverage the big data that insurance companies generate to enable transformational changes. Insurance providers continuously seek to manage risks as they offer different types of premiums to diverse customers. As for-profit organizations, insurance companies must ensure that revenue growth is supported by clarity when they underwrite policies, process claims, and expand their services.
Generative AI, proven to improve productivity in other industries, is in an excellent position to effect similar changes for insurance providers. Industry leaders are confident that insurance companies will explore generative AI in the coming months and potentially scale AI applications organization-wide if they can iron out the initial uncertainties.
Several insurance companies have taken the lead with generative AI usage. Liberty Mutual, a US insurer, developed an AI-based system that compares anonymized claims photos to estimate claims filed by vehicle owners. Meanwhile, SWICA, a healthcare insurer, developed a chatbot capable of addressing customers' queries and updating their personal details.
Benefits of AI in Insurance
Whether reducing claim processing time or strengthening insurance providers’ resilience against fraud, generative AI provides the necessary means when properly adopted. I share several examples below.
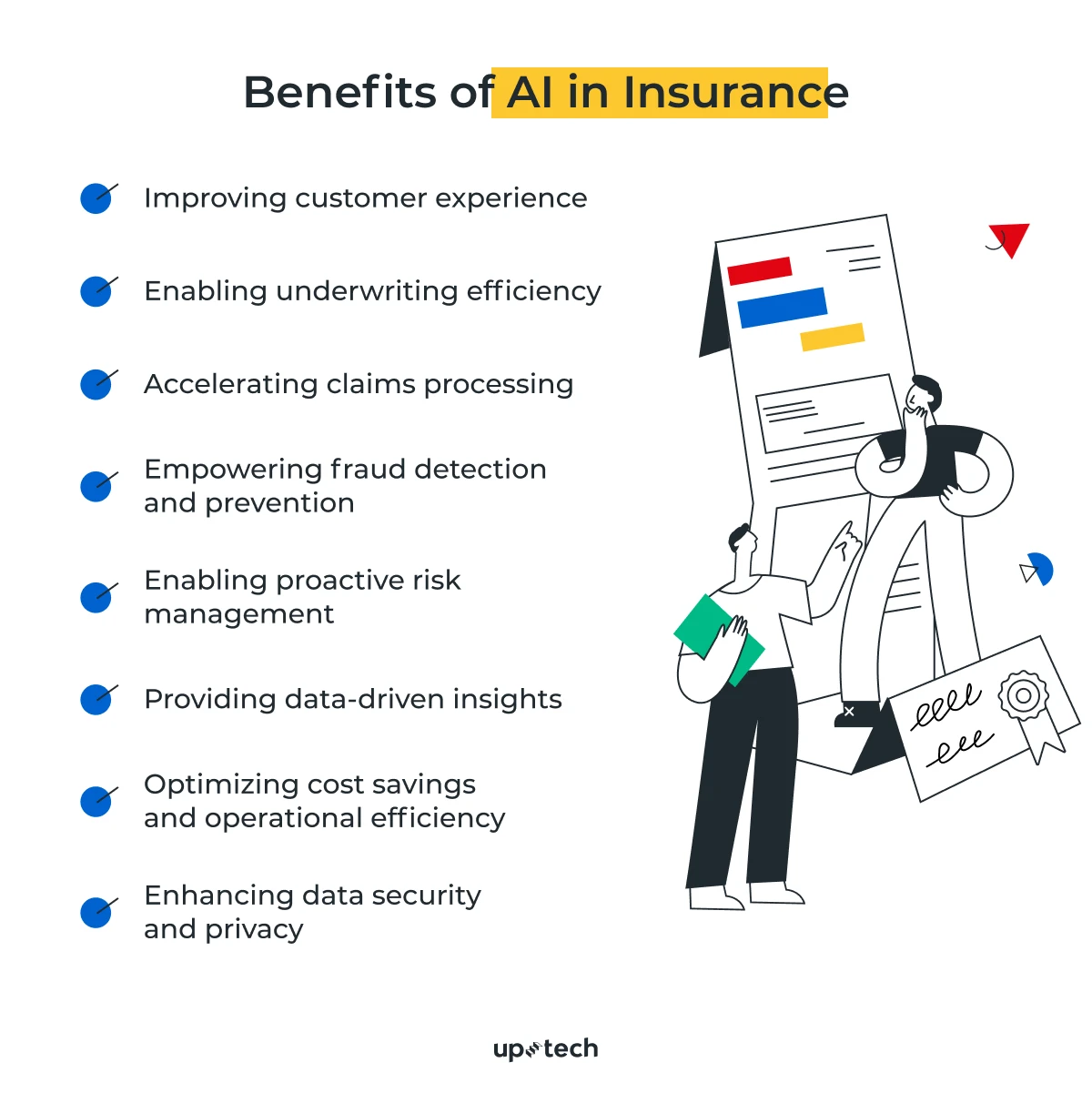
Improving customer experience
Customer engagement is crucial to retention and revenue growth in a competitive market. Generative AI can enable insurance companies to provide more responsive and personalized interactions when integrated with front-facing platforms. It lets customers generate policy summaries by asking conversational questions to the AI chatbot.
Enabling underwriting efficiency
Underwriters are tasked to create policies that attract customers while considering the risks that insurers bear. Conventionally, such endeavors are complicated by overwhelming data that human underwriters need help to process. With AI-generated predictions, underwriters can confidently estimate risks without sacrificing efficiency.
Accelerating claims processing
Manual intervention along the claim processing workflow can introduce bottlenecks that cause customer frustration. Furthermore, menial tasks, such as data entry, took up precious time from the insurance workforce. With sufficient training, generative AI can automate specific repetitive processes, leading to faster and more accurate claims.
Empowering fraud detection and prevention
Insurance companies face increasing threats that seek to jeopardize the integrity of their business model with fake claims and unethical practices. Generative AI safeguards against fraudulent claims by detecting suspicious patterns in data gathered from various customer interaction points. Then, the AI system would warn the appropriate personnel to prevent bad actors from profiting from such acts.
Enabling proactive risk management
AI helps insurance companies stay on the front foot when navigating risks the industry poses. For example, deep learning models can analyze demographic risk factors from historical data and predict risk factors that human analysts might overlook. This allows insurers to mitigate risks by preempting changes in market sentiments.
Providing data-driven insights
Global insurers sit on enormous data repositories that they can tap into to better understand their market, competitors, and customers. Generative AI provides the key to analyzing these data at scale, uncovering trends that help insurers chart and refine their strategies. Amongst the possibilities, generative AI can discover customer behaviors that help insurance marketers set up personalized campaigns.
Optimizing cost savings and operational efficiency
Insurers striving to reduce operational expenses and improve productivity will benefit from AI's automation and data analytics capabilities. Particularly, using AI automation and analytics helps insurers minimize resource wastage and accelerate decision-making.
Enhancing data security and privacy
Insurers can strengthen efforts to comply with data security and privacy regulations by integrating generative AI into existing workflow. Generative AI can analyze transactions and data access in real time, providing alerts when adversarial threats are detected. Besides, insurers can also analyze regulatory requirements with AI and compare them with the institution’s policies to ensure compliance.
Possible AI Use Cases in Insurance
The gradual adoption of generative AI solutions will positively transform workflows that insurers are accustomed to. Below are several practical applications of the technology in insurance.
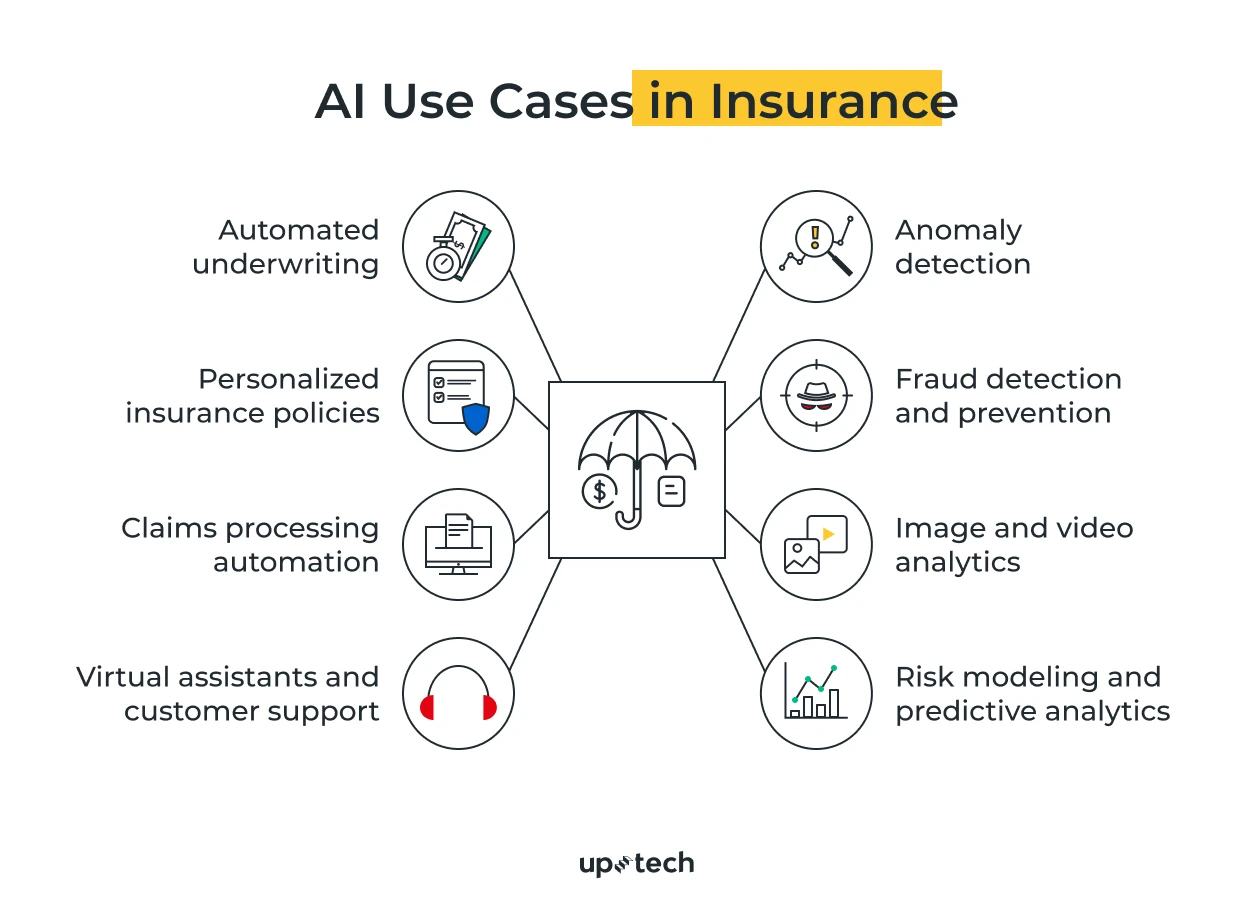
Automated underwriting
Generative AI can augment underwriters by automating processes such as risk assessment, premium estimation, and legal terms determination. For example, a medical insurer analyzes a patient’s medical history, age, earnings, and other demographic data before deciding the most optimal policy it can offer.
Personalized insurance policies
Generative AI helps insurance companies reinvent their market offerings with policies tailored to individual needs and risk factors. For example, a pet insurer compiles and analyzes spending behaviors, pet caring needs, types of pets, and other variables to create an attractive policy for pet owners.
Fraud detection and prevention
Generative AI prevents insurance companies from falling prey to bad actors with pattern detection capabilities. For example, a medical insurer uses AI to validate claims by ensuring all submitted bills and health reports are authentic.
Claims processing automation
Generative AI enables risk managers, underwriters, insurance analysts, and other specialists to automate repetitive or low-value work. For example, property insurance companies apply AI to automatically file submitted claims according to reported damages, location, and claim values.
Virtual assistants and customer support
Generative AI provides an interactive and responsive customer experience, improving retention and satisfaction. For example, auto insurance providers integrate their apps with a generative AI chatbot that assists policyholders in tracking claim disbursement.
Risk modeling and predictive analytics
Generative AI helps insurance providers prevent financial loss by forecasting undesirable scenarios with data analytics in advance. For example, travel insurers can apply generative AI modeling to predict natural or people-made incidents in specific countries before insuring travelers.
Anomaly detection
Generative AI is equally helpful in spotting suspicious trends that insurers would be alerted to. For example, auto insurers use AI to detect accident claims with contradictory evidence by analyzing and logically deducing data extracted from the submitted files.
Image and video analytics
Generative AI supports efforts in analyzing damages captured in images or video submissions to provide an accurate and timely assessment. For example, property insurers use deep learning models to analyze damages a building suffered to estimate the appropriate claim amounts it should disburse.
Tech Stack for Generative AI in Insurance
Unlike typical digital solutions, generative AI platforms heavily rely on machine learning and neural network algorithms. In this discipline, there are various methods, machine learning models, and efforts that you can apply to build AI-based insurance products.
Deep learning models
The recent breakthrough in machine learning resulted in AI models applying transformers and other neural network networks at scale. Deep learning models feature multiple hidden layers that extract more information from training datasets, allowing them to learn faster and more accurately than their predecessors. By representing word tokens into numeric representations called embeddings, the model can perform various natural language processing tasks, such as prediction, text generation, and classification.
For example,
- GPT-3.5, GPT-4, Bard, and LLaMa are AI models capable of understanding syntactic and semantic relationships of words like humans do. They are helpful in document categorization, fraud detection, and claims summarization for insurance providers.
- OpenAI Whisper is architected with simple encoder-decoder arrangements, allowing it to function as a speech recognition and machine translation engine. Insurers may find Whisper helpful when developing AI virtual assistants for conversing in multiple languages.
- DALL.E and Segment Anything Model (SAM) are deep learning models trained for image generation and processing. For example, auto insurers can use SAM to detect damages that vehicles suffer in the submitted photos automatically.
Machine learning algorithms
Prior to deep learning models, data scientists use machine learning architecture that doesn’t consume as much computing resources. While these AI algorithms are simpler, they are equally helpful in certain insurance use cases.
- Convolutional neural network (CNN) uses a series of convolutional filters that enable it to capture spatial representations effectively. In simpler words, insurers can use CNN to develop AI solutions for image or video analysis.
- Recurrent neural networks (RNN) enable representational learning by extracting key features in textual data. It is suitable for use cases like predictive modeling and sentiment analysis, such as assessing customer satisfaction from survey data.
- Variational autoencoder maps input to a probabilistic latent space, which it uses to reconstruct new data that resembles the distributional pattern. As such, the model is ideal in applications like anomaly detection because it doesn’t require a large amount of data to train.
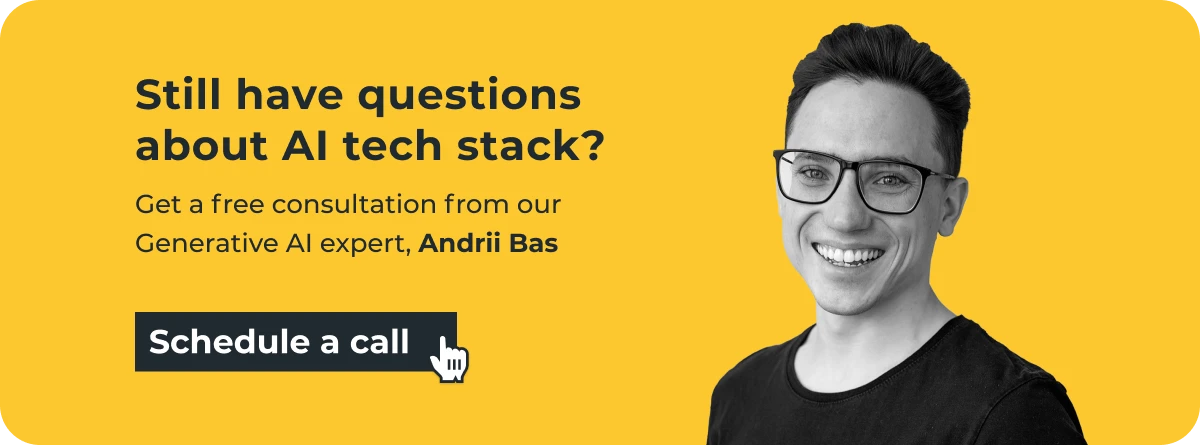
AI training
Regardless of which AI model or algorithm you use, they require training, fine-tuning, and continuous refinement to operate efficiently. Machine learning models use supervised and unsupervised training to develop inference capabilities. Meanwhile, larger models like GPT and Bard train unsupervised with unlabeled datasets but can be further fine-tuned for downstream tasks.
During unsupervised learning, large language models are fed large volumes of unlabelled datasets curated from diverse data sources. Without human guidance, the model uses techniques like clustering to find similar patterns in the data. This helps insurers develop AI systems capable of classifying claims, assessing customer sentiments, and detecting fraud.
Unlike simpler machine learning models, deep learning algorithms like GPT are capable of one or few-shot learning. Few-shot learning means the model can develop a sufficient understanding of a new topic with limited examples. This is possible because deep learning models bear significantly more complex architecture and are pre-trained with exceptional language understanding ability.
Challenges of Generative AI
Generative AI holds promises to unlock new opportunities for insurance providers, but there are challenges that you must be mindful of.
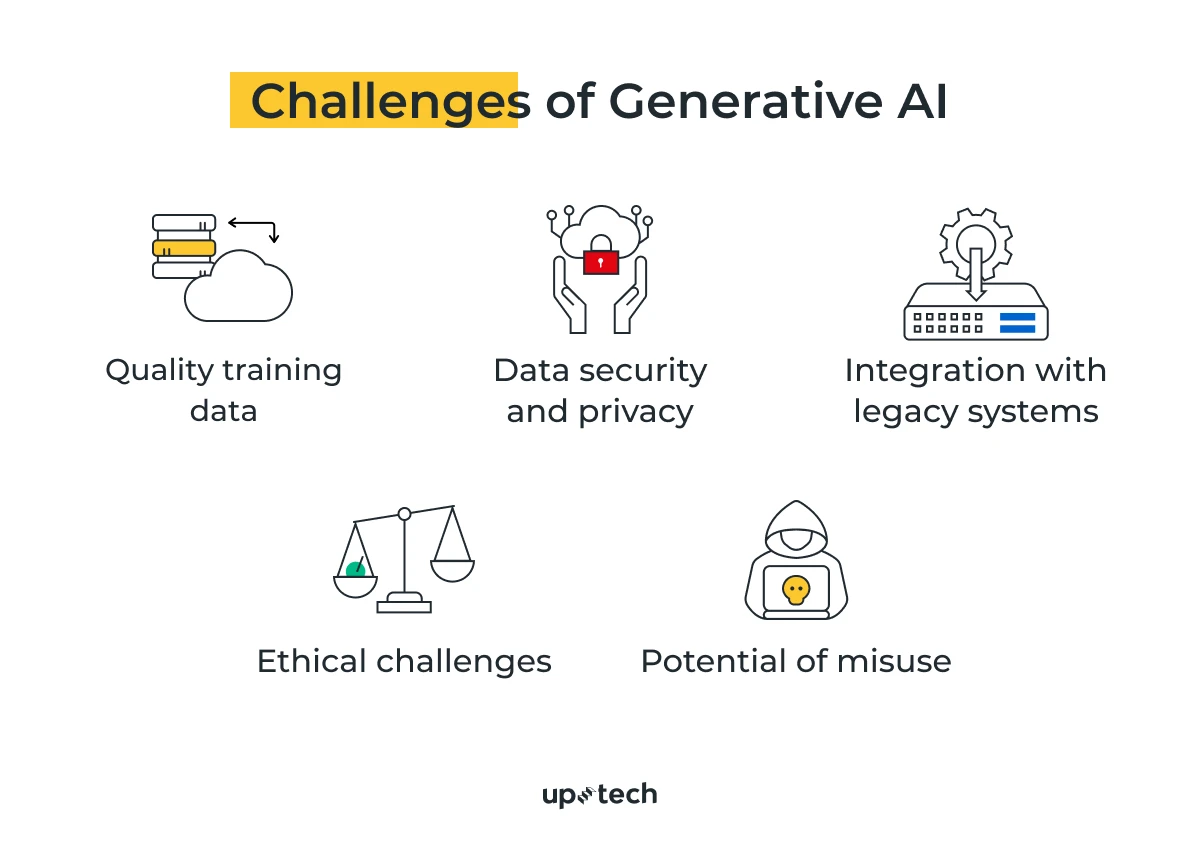
Quality training data
General machine-learning models cannot operate reliably without fine-tuning. For example, ChatGPT is an exceptional chatbot for linguistic tasks but was not trained with data specific to the insurance industry. As such, the model might produce inaccurate predictions that affect the decisions affecting policyholders.
To train or fine-tune models for insurance use cases, you must curate quality training data consisting of needed records or insurance claims, and other related documents. This proves challenging because not all insurance companies retain or have access to sufficient training data.
Data security and privacy
Developing AI solutions for insurance use cases involves acquiring, storing, and managing large volumes of sensitive information. There are concerns that inadequate security measures might lead to data breaches. As insurance is a business that operates on trust, you must deploy appropriate measures to safeguard data that models use to train and infer.
For example, insurance companies must encrypt data at all storage and exchange points. Proper identification and authorization mechanisms also help to prevent unauthorized access to confidential data. Moreover, such moves help insurers comply with regulations like HIPAA and GPDR when adopting generative AI.
.webp)
Ethical challenges
AI providers have acknowledged the limitations that deep learning models currently face. When predicting beyond the knowledge they train on, models can hallucinate, generating inaccurate results that people may mistake as facts. Without safeguards, insurers may act on these flawed results, affecting customer experience and legal outcomes.
Machine learning experts are equally concerned that deep learning models may exhibit societal bias and harmful behavior in certain circumstances. For example, the model may unfairly suggest differing claim amounts based on a specific race or gender. Such issues usually result from the training data the model learned from or the architecture of the neural network itself.
Integration with legacy systems
Some insurance companies operate with IT systems incompatible with the data, networking, and computing requirements that AI models need. This might cause some insurers to hesitate when introducing generative AI because it requires upgrading or replacing existing servers, software, and networking equipment.
Potential of misuse
Generative AI is a double-edged sword. Bad actors might misappropriate the technology to submit fraudulent claims that insurers pass off as genuine. For example, AI can produce realistic images depicting property damages to deceive insurers.
Tips on using Generative AI in Insurance
Like any emerging technologies, some challenges might hinder industry-wide adoption. However, don’t be discouraged by such challenges. Instead, use these tips to navigate technicalities and ethical challenges when developing your AI-powered insurance products.
- Curate training data from insurance claims, policies, personal records, and other sources that fairly present the target market's demographics. It helps to reduce bias and improve the model performance.
- Secure the data with appropriate security measures to comply with regulatory guidelines and protect customers’ interests.
- Choose a model appropriate to the intended task. For example, use a CNN instead of an NLP model to analyze image property damages.
- Apply safeguards to review arithmetic calculations that the AI model made. Note that language models are not designed for mathematics. They might produce erroneous results that affect claim disbursement if left uncorrected.
- Continuously fine-tune or refine the model with anonymized gathered from user interactions with the AI system. Doing so allows the model to train and improve on key performance metrics.
How can Uptech help?
Uptech is an international app development company that serves clients worldwide. We help startups, businesses, and enterprises solve real-world problems by delivering solutions that users love. Our team is also at the forefront of the AI race, with a stellar record of developing and integrating generative AI technologies for various industries.
Our recent effort is Hamlet, an app that creates concise and precise summaries from uploaded text and PDFs. We deployed a language model within a web app and incorporated UI/UX features to provide an intuitive user experience. Our team has also successfully integrated a generative AI assistant for Plai, which allows HR managers to create recommendations for employee growth plans.
.webp)
Developing digital products for the insurance industry requires careful deliberation of regulations protecting customer data and privacy. We can draw from our experience building a mental health app that meets stringent requirements in the US.
At Uptech, we believe in bridging your technological needs while balancing cost and time to market. Our team can deliver a proof-of-concept in typical circumstances for $20k in 1 month.
As our client, Angler AI, puts it, “Uptech delivered the tasks on time and ensured excellent communication.”
.webp)
Summary
Generative AI is a major technological force that will drive transformational changes within the insurance industry. It promises capabilities to bring efficient underwriting, risk assessment, claims processing, and more in a tightly governed industry. Insurance agents, underwriters, risk managers, and other workforce serving policyholders will benefit from AI's automation, predictive analytics, and other features.
I’ve highlighted the major concerns you should consider when developing AI products for the insurance industry. Also, I’ve shared tips that help you mitigate known challenges and create a more accurate AI system. Still, it’s more strategic to partner with an AI app developer with a field-proven track record.
Drop us a message, and let’s start bringing your ideas to life.