From a mere 840,000 telehealth visits in 2019 to a whopping 52.7 million in 2020, the healthcare landscape has witnessed a transformative embrace of technology. Such a significant adoption of virtual healthcare assistant highlights a tangible shift as institutions aim to optimize patient experiences and streamline administrative tasks. Patient satisfaction rates are rising with these AI-driven tools' enhanced accuracy, responsiveness, and 24/7 availability.
In the pharma sector, when we talk about AI, it's not just a nod toward better patient care. It's also about significant business gains. Having worked closely with cutting-edge platforms at Uptech, where I'm the co-founder and tech lead, I've seen the transformative power of tools like Dyvo.ai and Plai.team's AI assistant, Angler.AI, Tired Banker, and Hamlet. The potential of AI is vast. Embracing AI-driven strategies can greatly improve clients' Lifetime Value (LTV), blending profitability with unmatched value.
Pharma companies are rapidly identifying the merits of these technological gems, eager to harness tools that guarantee unparalleled patient engagement while simultaneously boosting their financial outcomes. The ideal blend of superior healthcare and thriving business marks the zenith of today's AI-powered app development trajectory, promising a luminous tomorrow.
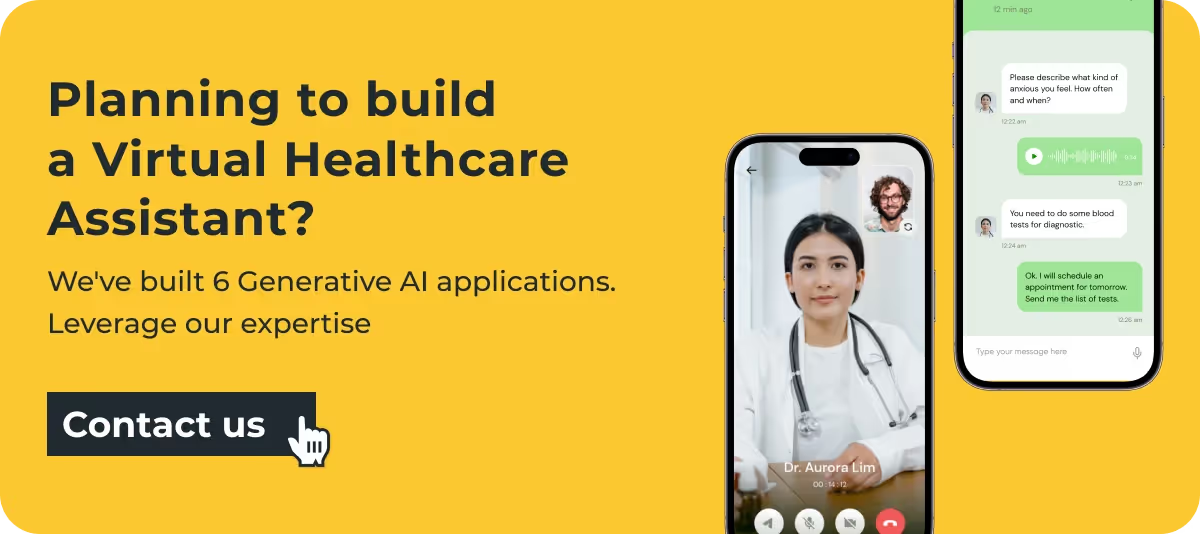
What Exactly is a Virtual Healthcare Assistant?
A Virtual Healthcare Assistant, at its core, is an AI-powered digital tool designed to transform the patient-care experience. Imagine a sophisticated software platform that understands your health concerns and offers immediate, personalized guidance. That's the marvel of a Virtual Healthcare Assistant.
So, who's capitalizing on this digital wonder? The user spectrum fetches far from large-scale hospitals to solo health practitioners and individual patients. A staggering report from Softweb Solutions hints at the massive adoption wave: Artificial intelligence in the healthcare market is projected to soar to USD 36.1 billion by 2025. And let's remember real-world implementations that are reshaping the healthcare industry.
Why Virtual Health AI Assitant Is a Game-Changer
AI-driven Virtual Healthcare Assistants (VHAs) stand tall as a beacon of progress in medical innovations. They are transforming how care is delivered and how patients and professionals engage with the broader health system. But what exactly makes them the show-stealers in the healthcare world? Below is the detailed answer to this query.
1. A Gateway to Unparalleled Accessibility
Gone are the days when accessing health advice meant scheduling appointments and waiting in long queues. With VHAs, healthcare guidance is but a click away. For instance, Saal.ai's cognitive virtual assistant offers a bilingual platform ready to provide personalized health insights anytime, anywhere. The upshot? Prompt medical attention and proactive health management.
2. Scalability Tailored to Your Needs
AI-powered virtual assistants in healthcare adapt for everyone, from sprawling healthcare facilities to solo practitioners. Their flexible architecture ensures that healthcare professionals can upscale or downscale services based on patient influx or specific requirements. It allows dynamic resource allocation and patient management.
3. Riding High on Virtual Medical Trends
With the ongoing trend of telemedicine and virtual consultations, VHAs fit right in. These assistants complement and strengthen the virtual health wave, ensuring patients experience seamless digital medical consultations.
4. Training That Rivals the Best
Virtual healthcare assistants aren't just coded programs but are embedded with specialized medical training. This ensures that the advice dispensed and the patient queries addressed are on par with what trained medical professionals would offer.
5. Your Health Journalist
VHAs excel at chronicling health metrics. Be it tracking a patient's blood pressure fluctuations over a month or monitoring glucose levels, these digital allies ensure that individuals remain informed about their health trajectories.
6. Liberating the Human Hands
One of the significant advantages is resource optimization. Virtual assistants in healthcare shoulder responsibilities like patient query resolution and appointment reminders. So, medical professionals focus on intricate tasks, reducing burnout and ensuring better patient care. Consequently, facilities can manage resources more efficiently, streamlining operations.
7. Crafting a Personalized Health Odyssey
Each patient's health journey is unique. Recognizing this, virtual nursing assistants like those developed by Dyvo.ai integrate features like gamification to engage users better. By providing a tailored experience, patients are more invested in their health, leading to better outcomes.
8. Minimizing the Age-Old Human Error
Even the best professionals can have an off day, leading to minor oversights. However, in healthcare, these can have significant repercussions. Virtual assistant healthcare, with its data-driven algorithms, ensure consistent accuracy, significantly reducing the chances of human errors.

Tackling the Hurdles of AI-driven Virtual Healthcare Assistants
As promising as AI-driven virtual healthcare assistants are, the journey to flawless integration into the healthcare industry is challenging. These revolutionary platforms depend heavily on the precision of data and the appropriateness of the algorithms that power them. Below, I discuss the significance of these challenges and offer insights into their potential implications.
The Imperative of the Right Database
Data is the backbone of any reliable AI system, especially in the sensitive field of healthcare. Generative AI, the technology behind many virtual healthcare assistants, feeds on vast datasets to produce predictions, suggestions, and solutions. This means that every piece of medical data – from simple symptoms to complex imaging studies – becomes a fundamental building block for these systems.
Imagine an AI-driven virtual healthcare assistant advising a patient based on slightly skewed medical records. Such misinformation can lead to the assistant suggesting incorrect treatments or missing out on crucial medical interventions. The aftermath? This is a significant risk to the patient's health due to erroneous data.
Therefore, the essence of accurate output is deeply rooted in the purity and completeness of the datasets used. Only when healthcare providers and patients share an unshakeable trust in this technology can it be integrated seamlessly into medical care?
The Essentiality of the Right Algorithm
Data is just one piece of the puzzle. The algorithm that processes this data is equally pivotal. Suppose an algorithm is nurtured on a dataset that doesn't mirror the diversity and depth of the actual population. In that case, it can churn out outputs that are not just inaccurate but can also be detrimental.
Consider a hypothetical AI model trained predominantly on data from a particular demographic. Suppose a patient from a different demographic seeks advice from this AI system. In that case, there's a high probability of the guidance needing to be better suited, leading to potential health risks for the patient.
Addressing these algorithmic biases is not just a technical necessity; it's a moral imperative. Ensuring the ethical use of AI in healthcare is non-negotiable. By refining the algorithms and continuously improving their training datasets, we can work towards a future where AI-driven healthcare assistants reliably enhance healthcare outcomes, putting patients' well-being at the forefront.
Balancing AI and Ethics in Healthcare
The rise of generative AI in healthcare comes with its own set of ethical dilemmas. One of the primary concerns surrounds the use of sensitive patient data. Is using personal health records, treatment details, and other intimate data to train an AI ethically? While the aim is to refine the system for improved care, data misuse's always questioned.
Additionally, the specter of bias within algorithms persists. If AI systems aren't trained with diverse datasets, they could inadvertently favor one demographic over another. Such preferences may not just lead to unequal healthcare access but also to disparities in treatment outcomes. Despite these challenges, there's a silver lining. AI, being devoid of human emotions, offers a judgment-free zone. Patients might find solace in discussing their issues without fearing judgment or stigmatization, something they might hesitate to do with human caregivers.
The Integration Challenge
While AI presents a groundbreaking approach to healthcare, its seamless integration into existing health systems remains complex. We're not just talking about syncing up software; it's about integrating an entirely new way of doing things without causing disruption. Healthcare professionals have their established workflows, and combining AI means ensuring these systems talk to each other efficiently and without hiccups. It's a colossal task that demands coordination, training, and sometimes a shift in mindset.
.avif)
Guarding the Fort: Security and Data Privacy
Generative AI's capability to churn through vast amounts of data is its strength and its Achilles' heel. As AI works through these heaps of sensitive patient data, the stakes for securing this data skyrocket. The potential for unauthorized access and misuse of patient data is a real and present danger. Imagine the ramifications if personal health records were manipulated or sold.
Navigating the maze of compliance adds another layer of complexity. With legislation like HIPAA in place, ensuring that every piece of data is handled with utmost care — both when stored and during transit — is not just best practice; it's the law. Ensuring full compliance while leveraging the full potential of AI is a tightrope walk that healthcare providers and tech developers must master.
5 Ways to Follow HIPAA while Building AI-powered Healthcare Tools
Healthcare technology, especially when dealing with Protected Health Information (PHI), necessitates adherence to stringent standards. One such standard, pivotal for healthcare apps, is the Health Insurance Portability and Accountability Act (HIPAA). This act ensures that any information, from an MRI scan to a simple diagnosis, remains protected and confidential.
One approach could be liaising directly with OpenAI, seeking a Business Associate Agreement (BAA), and defining how OpenAI handles PHI. However, for this to be feasible, having an Enterprise Agreement with OpenAI becomes a requisite, coupled with a legitimate use case. Should this not be an option, other solutions include:
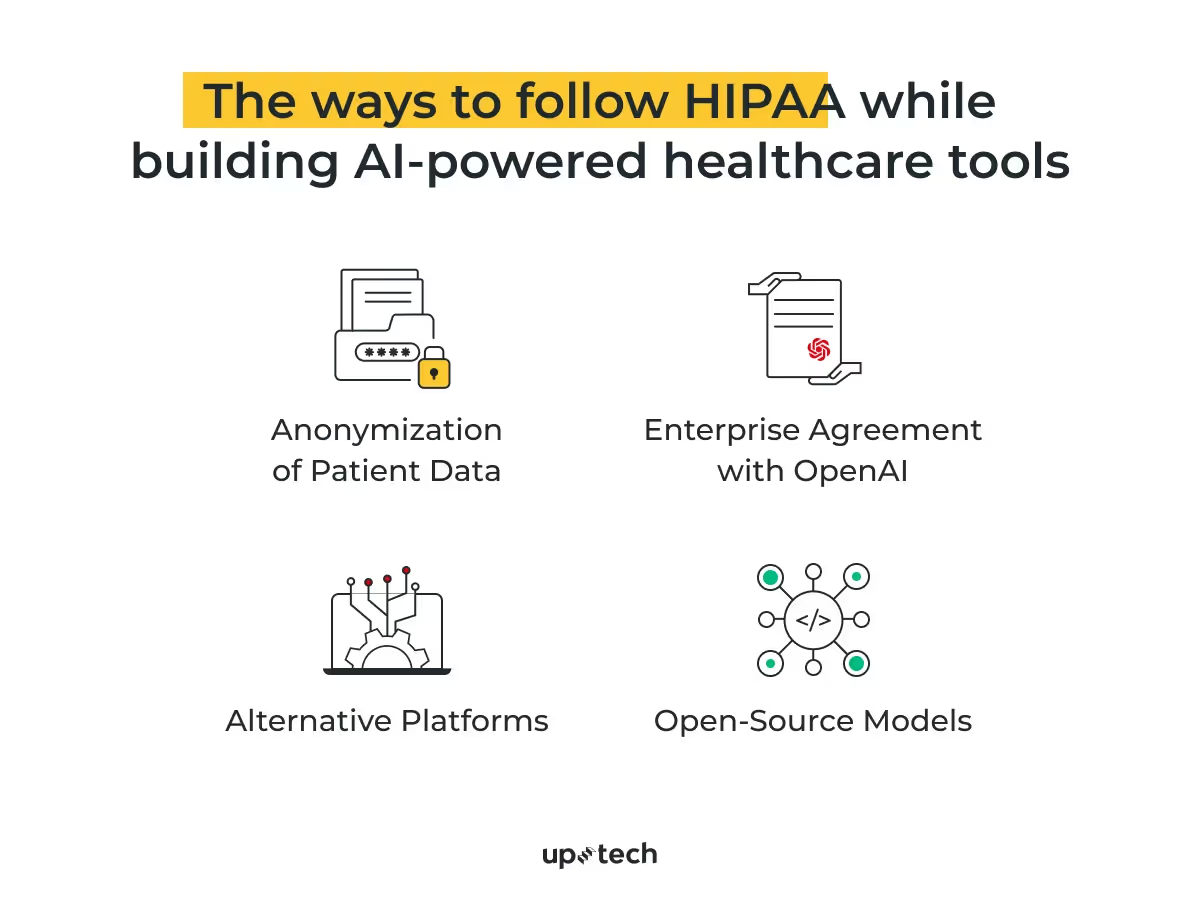
1. Hosting other models instead of ChatGPT In-House
Hosting AI models on your organization's servers, ensuring complete control over data and infrastructure.
- Advantages: Direct control over the model's environment allows for a tailored approach to security, aligning directly with HIPAA requirements. Reduced risk of data breaches as data remains within the organization's infrastructure.
- Considerations: Requires a robust IT infrastructure and expertise. Maintenance, uptime, and ongoing compliance are the organization's responsibility.
2. Anonymization of Patient Data
Transforming patient data to remove or obscure personal identifiers, makes it challenging to link data to individual patients.
- Advantages: Renders data useless to unauthorized parties, protecting against potential misuse in case of breaches.
- Techniques: Tokenization, data masking, and de-identification are common strategies.
- Considerations: Balancing data usability for authorized healthcare tasks with anonymization can be challenging.
3. Alternative Platforms
Utilizing other AI platforms that are specifically designed or better suited for healthcare data processing in compliance with regulations.
- Advantages: Platforms like Microsoft’s Azure Open AI Service have advanced security features. They may offer more extensive support and documentation for healthcare integrations.
- Considerations: Assess migration costs, compatibility with existing systems, and potential learning curves.
4. Open-Source Models
Utilizing AI models with publicly available source code allows for in-depth customization and scrutiny.
- Advantages: Complete transparency with the model's workings. Potential security flaws can be identified and rectified. Allows for bespoke modifications to meet specific HIPAA standards.
- Considerations: Requires expertise for effective modification and maintenance.
5. Enterprise Agreement with OpenAI
Forming a formal agreement with OpenAI that defines terms of use, responsibilities, and how PHI is managed.
- Advantages: A clear framework ensures both OpenAI and the healthcare organization understand their obligations, ensuring accountability.
- Process: Liaise with OpenAI's team, present a legitimate use case, and discuss agreeable terms.
- Considerations: This may involve legal reviews, and negotiations, and not all use cases might be accepted.
How do healthcare app developers navigate this?
One approach could be liaising directly with OpenAI, seeking a Business Associate Agreement (BAA), and defining how OpenAI handles PHI. However, for this to be feasible, having an Enterprise Agreement with OpenAI becomes a requisite, coupled with a legitimate use case.
Should this not be an option, other solutions include:
Anonymization of Patient Data: A widely accepted practice in many healthcare applications, anonymization ensures patient data is rendered unreadable, undecipherable, and essentially anonymous.
Alternative Platforms: Switching to platforms that offer better data privacy controls, like Microsoft’s Azure Open AI Service, can be a viable option.
Open-Source Models: By leveraging open-source Large Language Models (LLMs), developers can customize and deploy them in compliance with HIPAA stipulations.
Crafting With the Right Tools: The Tech Stack for AI Healthcare Virtuosos
In the realm of AI-driven virtual healthcare assistants, the choice of technology plays a pivotal role. This isn't just about ensuring the assistant functions correctly; it's about guaranteeing precision, efficiency, and compliance, especially when dealing with matters as delicate as health. The underpinnings of a robust virtual healthcare assistant are based on a combination of advanced AI models, sophisticated algorithms, and potent neural networks. Here's a brief breakdown:
AI Models:
The choice of AI models primarily dictates the performance of the healthcare assistant. Some of the renowned models in this domain include:
GPT 3-4: These encompass variations such as Davinci, Curie, Babbage, and Ada.
DALL.E: A neural network that generates images from textual descriptions.
Whisper: An automatic speech recognition system.
Embeddings, Moderation, Stable Diffusion, Midjourney, Bard, and LLaMA further enrich the capabilities of the AI system, tailoring responses and solutions according to patient needs.
Algorithms:
One must choose the right learning algorithms to ensure the AI understands and processes medical data proficiently. Here's a snapshot:
Supervised/Unsupervised Learning: These are the foundational stone for AI learning, where algorithms are trained with labeled or unlabeled data.
Clustering: For grouping patient data or symptoms.
Metric Learning and Fewshot Learning: Essential for understanding similarities and differences in vast datasets.
Manifold Learning: For capturing the essence of high-dimensional data.
Neural Networks:
These are the crux of the AI system, driving the actual processing of information:
CNN (Convolutional Neural Networks): Excellently suited for processing medical imaging data.
RNN (Recurrent Neural Networks): Great for sequences like a patient's historical data.
Representation Learning, Manifold Learning, Variational, Autoencoders, and Bayesian Networks are pivotal for deciphering patterns, while Autoregressive Networks predict future patient outcomes based on historical data.
A Step-By-Step Blueprint to Craft Your AI Virtual Healthcare Assistant
Establishing an AI-driven healthcare tool needs meticulous steps. Let’s explore the process, from defining its core purpose to ensuring sustained efficacy and relevance in the dynamic medical landscape.
1. Define the Purpose
Before diving into the technical aspects, it's vital to delineate the specific roles you want the virtual healthcare assistant to play. Are you aiming for a solution that provides preliminary diagnoses based on patient symptoms? Or perhaps a tool that assists in managing and monitoring chronic conditions like diabetes mellitus or hypertension? Defining the exact purpose will steer the subsequent phases in the right direction.
2. Collect and Prepare Data
For any AI-driven solution in healthcare, the essence lies in the quality and relevance of data. Procure diverse datasets, ensuring they encompass a broad range of medical scenarios. This could involve sourcing electronic health records (EHRs), imaging studies like MRIs or X-rays, and laboratory test results. But remember, while gathering patient-related data, always adhere to guidelines, ensuring data anonymization and adhering to HIPAA regulations.
Data Cleansing: The data must undergo a rigorous cleansing process once collected. This involves filtering out anomalies, ensuring no missing data, and structuring it aptly for training.
Data Annotation: In healthcare, annotation becomes crucial. For instance, in radiology, clearly demarcating pathologies on imaging scans ensures the ML model comprehends what to identify.
3. Develop a Machine-Learning Model
Here's where medical knowledge marries technology. Choose an algorithm or set of algorithms suitable for the medical tasks at hand. For diagnostic tools, classification algorithms might be apt. Regression models could be more fitting if you aim to predict disease progression. And for tasks that involve image recognition, like spotting tumors in radiology images, convolutional neural networks (CNN) would be ideal.
4. Train the ML Model
Once the machine learning model is set, it's time to feed it the prepared data. This training phase allows the model to learn from the data, fine-tuning its internal parameters to make accurate predictions or classifications. Regularly validate its performance using a subset of data, ensuring it’s not just memorizing (overfitting) but genuinely understanding medical patterns. Utilize medical metrics like sensitivity, specificity, and positive predictive value to gauge its performance accurately.
This iterative process might require several rounds of tweaking the model to achieve optimum accuracy, especially when dealing with intricate medical conditions.
5. Add the Assistant to Your AI Service
After training, it's time to integrate the model with an assistant framework, the interactive layer between the AI and the end users. Platforms like Microsoft's Azure Bot Service or AWS Lex are notable options considering their robustness and scalability. When embedding, ensure that the chatbot is equipped to understand and process medical jargon, terms, and even potential patient misspellings or colloquialisms.
Learn more about chatbots in healthcare in our blog.
6. Curate a User Interface
The efficacy of a virtual healthcare assistant isn't just about accuracy; user experience plays a pivotal role. Design an interface that's intuitive and user-friendly. Working alongside clinicians to ensure the interface aligns with medical workflows is beneficial, minimizing the learning curve. Include features like voice recognition, especially considering older people or those with motor disabilities. This holistic approach ensures the AI tool is accessible to a broad patient demographic.
7. Test Your Virtual Health Assistant
Before the full-scale launch, a pilot phase is crucial. In this testing phase, engage real-world users, medical professionals, and potential patients. Collect feedback, paying special attention to false positives or negatives in medical predictions. Ensure the tool adheres to clinical guidelines and doesn't misguide users inadvertently.
8. Launch the AI Model
Now is the time to roll out the virtual healthcare assistant to the broader audience. It's advisable to have medical professionals on standby during the initial phase, ensuring that any medical queries or concerns raised by users are promptly and accurately addressed.
9. Monitoring and Upgradation
The world of medicine is constantly evolving, with new research, findings, and guidelines emerging regularly. As such, the AI model shouldn't be static. Periodically retrain it with fresh data, ensuring it remains updated with the latest medical knowledge. Furthermore, continually assess user feedback and integrate any recommended enhancements from a medical and user-experience perspective. It's also pivotal to look for emerging cyber threats, reinforcing the tool's security protocols to safeguard patient data.
Essential Features Every AI Healthcare Assistant Needs
The features of your virtual assistant can make a stark difference between a good tool and an exceptional one. Infused with the right parts, an AI healthcare assistant can cater to patient's immediate needs and anticipate and address potential health concerns. Let's explore the vital features that an AI-driven healthcare assistant must possess:

1. Medical Information
Providing accurate, evidence-based medical information is crucial. Users depend on these assistants to clarify doubts, understand treatments, and learn about conditions. Consider a patient newly diagnosed with diabetes. The AI assistant can provide them with comprehensive information about the disease, dietary guidelines, and lifestyle modifications to manage the condition better.
2. AI-Personalization
Every patient is unique, and so should their care. AI personalization ensures that the assistant tailors' advice and information to each user's medical history and preferences. Hence, if a user frequently asks about cardio exercises, the AI assistant can personalize suggestions by recommending heart-healthy diets or new cardiovascular workouts.
3. Reminders and Alerts
Consistency in medical regimens is vital. An assistant should be able to send reminders for medication, upcoming doctor appointments, or routine check-ups. For elderly patients on multiple medications, the AI system can send timely alerts, ensuring they don't miss a single dose, thereby preventing potential health complications.
4. Symptom Diagnosis
An initial analysis based on symptoms can be invaluable. While not replacing doctors, the AI tool can offer possible explanations or suggestions based on symptom inputs. A user might input symptoms like fever, fatigue, and joint pain. For example, the AI assistant can suggest these might indicate conditions like flu or dengue, recommending an immediate medical consultation.
5. Mental Health Support
Mental health is as crucial as physical well-being. The assistant should offer tools, resources, or immediate assistance options for mental health concerns. So, if a user expresses chronic sadness or despair, the AI assistant can recommend therapeutic relaxation exercises or contact mental health professionals.
6. Connection with Wearable Devices
With the advent of wearable health tech, integration with these devices can provide real-time health insights. If a smartwatch detects an irregular heart rhythm, the AI assistant can analyze the data, provide preliminary feedback, and suggest visiting a cardiologist if required.
Tips to Maximize Benefits of Generative AI in Healthcar
Leveraging the power of Generative AI in healthcare can be a game-changer. However, to harness its full potential, certain best practices are paramount. Below are key strategies to ensure optimal and safe usage:

Prioritize Data Protection: Always safeguard data meticulously. HIPAA compliance is non-negotiable when dealing with personal health information (PHI). As healthcare professionals, the sanctity and confidentiality of patient data must be upheld.
Quality Over Quantity in Data Collection: The precision of Generative AI hinges on the quality of datasets used, encompassing medical records, lab outcomes, and radiographic evaluations. Imagine the ramifications of AI misinterpreting an echocardiogram due to an incomplete dataset. Such lapses compromise patient trust and can have grave implications on health outcomes.
Verification is Vital: While AI's prowess is undeniable, it isn't infallible. If the AI model provides cardiac medication dosages or suggests specific treatments, validate these before application. Treat AI as an advisor rather than an absolute judge.
Selection of the Apt Algorithm: An algorithm's efficiency correlates directly with the dataset it's trained on. For instance, if an AI model, primed predominantly on pediatric data, is used for geriatric patients, the results could be less than optimal and dangerous. The algorithm's alignment with the target demographic is pivotal to preventing misdiagnoses and ensuring the best patient outcomes.
Continuous Refinement: Deployment isn't the endgame. Iteration is intrinsic to the AI journey. Garner real-time data, adhering to legal boundaries and ensuring it's anonymized. When fed back, this continuous influx of information refines the AI model, enhancing its diagnostic acumen and therapeutic recommendations.
Unlock Your Healthcare Catalyst with Uptech
At Uptech, we don't just understand technology; we know its transformative power in healthcare. Our prowess is showcased in our creation: the Mental Health App. This sophisticatedsophisticated platform enhances patient experiences and outcomes.

Beyond this, we integrated an advanced AI assistant into Plai, exemplifying our expertise in melding AI with real-world applications. Our elegant yet robust approach means we can develop a Proof of Concept (PoC) in one month, all at a cost-effective price of $20K.
Uptech isn’t just another tech company; we are partners in pioneering AI-driven healthcare solutions. Together, let’s chart the future of patient care.
Summary
The advancement and integration of AI into the healthcare realm have opened new avenues for patient care, but it's crucial to approach this evolution with diligence and expertise. Here's a recap of the pivotal considerations we've discussed:
- The cornerstone of AI's efficacy lies in quality data and representative algorithms.
- Ethical considerations, especially data privacy, and adherence to HIPAA guidelines, are paramount.
- A strategic tech stack incorporating state-of-the-art AI models, neural networks, and algorithms ensures robustness.
- Feature prioritization, from AI-personalized insights to wearable device integration, enhances user experience.
- While generative AI is transformative, it requires continuous iterations and real-time improvements.
- Uptech is a testament to innovative healthcare solutions, showcasing the possibilities when technology meets healthcare.
To explore this transformative journey further and understand how AI can be tailored to your healthcare endeavors, connect with our dedicated team.